Business analytics is the process of using data to improve business decisions. It involves collecting, cleaning, and analyzing data to identify trends, patterns, and insights that can be used to improve business performance.
The business analytics process is critical because it can assist businesses in:
- Increase revenue and profitability
- Reduce costs
- Improve customer satisfaction
- Make better decisions
- Gain a competitive advantage
Unlock your potential with Scaler’s comprehensive courses. Join now and start mastering the skills that will shape your future.
Benefits of Following a Structured Approach:
Adopting a structured approach to business analytics offers numerous advantages, enabling organizations to:
- Define their goals and objectives
- Collect Appropriate Data
- Analyze the data effectively
- Make data-driven decisions
- Measure the impact of their decisions
A structured approach can also help businesses to avoid common pitfalls, such as:
- Collecting the wrong data
- Analyzing the data incorrectly
- Making decisions based on incomplete or inaccurate data
Step 1: Defining the Business Needs
The foundation of any successful business analytics process lies in clearly defining the business needs. This involves a thorough understanding of the company’s objectives, identifying the core problem to be addressed, and setting measurable targets that will guide the analysis and decision-making process.
Identifying Business Objectives and Goals: Begin by coordinating with the overall business strategy. What are the company’s key priorities? Do they want to increase revenue, cut costs, improve customer satisfaction, or enter new markets? Engaging with stakeholders across different departments is crucial to gaining a holistic view of the business goals.
Understanding the Problem to be Solved: Once the broad objectives are established, it is time to focus on the specific problem that business analytics seeks to solve. Is it a decline in sales, high customer churn, inefficient operations, or something else? Clearly articulating the problem helps in framing the right questions and focusing the analysis efforts.
Setting Clear and Measurable Targets: To measure the success of the business analytics process, it’s essential to define measurable targets. These could include Key Performance Indicators (KPIs) such as revenue growth, customer retention rate, cost reduction, and market share. Setting specific targets provides a benchmark against which the results of the analysis can be evaluated.
Step 2: Exploring the Data
After defining the business requirements, the next critical step in the business analytics process is data exploration. This involves gathering all relevant data sources, cleaning and preparing the data for analysis, and conducting initial explorations through visualization and summary statistics.
Collecting Relevant Data: Based on the defined business objectives and problem statement, identify the necessary data sources. These could include internal databases, customer surveys, marketing analytics tools, social media data, and external market research reports. Ensure the collected data is comprehensive and representative of the problem at hand.
Data Cleaning and Preparation: Raw data is frequently messy, with errors, inconsistencies, missing values, and outliers. Data cleaning involves addressing these issues to ensure the accuracy and reliability of the analysis. This could include removing duplicates, filling in missing values, standardizing formats, and addressing inconsistencies. Data preparation also involves transforming the data into a suitable format for analysis, such as aggregating, filtering, or deriving new variables.
Initial Data Exploration and Visualization: Once the data is clean and prepared, begin exploring it to gain initial insights. This could include calculating summary statistics such as mean, median, and standard deviation to better understand the data’s central tendency and distribution. Visualizing the data through histograms, scatter plots, or other charts can help identify patterns, trends, relationships, and potential outliers.
Step 3: Analyzing the Data
After cleaning and preparing the data, the next step is to delve deeper into the analysis. This involves applying various statistical methods to identify trends, correlations, and patterns that can shed light on the underlying business problem.
Applying Statistical Methods: Statistical analysis tools play a crucial role in deriving meaningful insights from data. Hypothesis testing, regression analysis, ANOVA, and clustering are used to validate assumptions, quantify relationships between variables, and identify groups or segments in data. The choice of statistical methods depends on the nature of the data and the specific questions being asked.
Identifying Patterns and Insights:
Patterns and correlations in data can be discovered through careful analysis. These might reveal hidden relationships between customer behavior and purchasing patterns, operational inefficiencies, or market trends. Identifying these insights is critical for understanding the underlying factors that influence business outcomes.
Using Analytical Tools and Software: Modern business analytics relies heavily on specialized software tools that simplify the analysis process. These tools, including R, Python, SAS, SPSS, and Tableau, provide a diverse set of statistical functions, data visualization capabilities, and machine learning algorithms. They enable analysts to efficiently process large datasets, automate repetitive tasks, and present findings in a clear and concise manner.
Step 4: Predicting What is Likely to Happen
In this phase, the focus shifts towards leveraging the analyzed data to forecast future trends and potential outcomes. This is where the true power of business analytics emerges, allowing organizations to make proactive decisions rather than simply reacting to past events.
Building Predictive Models: Predictive modeling involves creating statistical models that can estimate future values or behaviors based on historical data. These models employ a variety of techniques, including regression analysis, time series forecasting, decision trees, and even more sophisticated machine learning algorithms. The choice of model depends on the specific business problem and the available data.
Using Machine Learning Algorithms: Machine learning algorithms play a crucial role in predictive modeling. These algorithms can learn from data and detect patterns that humans may overlook, making them invaluable for discovering hidden insights and making accurate predictions. Common machine learning algorithms used in business analytics include linear regression, logistic regression, decision trees, random forests, and neural networks.
Evaluating Model Accuracy and Performance: Once a predictive model is built, it’s essential to assess its accuracy and performance. This entails testing the model on a separate dataset that was not used in training and comparing its predictions to the actual results. Various metrics, like mean squared error (MSE), mean absolute error (MAE), or accuracy, can be used to evaluate the model’s performance. Regular monitoring and refinement are crucial to ensuring that the models remain accurate and relevant as new data becomes available.
Step 5: Optimizing
The optimization phase is where the insights gained from analysis and prediction are translated into actionable strategies. This step entails using optimization techniques, testing various scenarios, and ultimately deciding on the most effective course of action to achieve the defined business objectives.
Implementing Optimization Techniques: Optimization techniques aim to find the best possible solution given a set of constraints. In the context of business analytics, this could mean increasing revenue, lowering costs, improving efficiency, or optimizing resource allocation. These techniques can range from simple linear programming to more complex algorithms like genetic algorithms or simulated annealing.
Scenario Analysis and Simulation: Scenario analysis involves exploring different “what-if” scenarios to understand the potential impact of various decisions on the business. This can be accomplished using simulation models that mimic real-world processes and allow for the testing of various strategies under different conditions. By simulating different scenarios, businesses can evaluate the potential risks and rewards of different choices and make more informed decisions.
Choosing the Best Course of Action: Based on the outcomes of optimization techniques and scenario analysis, the most promising course of action is chosen. This decision should be based on a combination of quantitative and qualitative data, such as the organization’s risk tolerance and strategic objectives. The chosen course of action should be aligned with the overall business goals and have the highest potential for achieving the desired outcomes.
Ready to become an expert in your field?
Scaler Courses offer expert instruction, hands-on projects, and career guidance to help you succeed.
Step 6: Making a Decision and Measuring the Outcome
This stage is the culmination of the business analytics process, in which insights and predictions are transformed into concrete actions. The previous steps’ findings heavily influence the decision-making process, and the outcome measurement ensures that the chosen solution is effective and aligned with the business goals. From the previous steps, while the outcome measurement ensures that the chosen solution is effective and aligned with the business goals.
Decision-making based on analysis and prediction: With a thorough understanding of the data, predictive models, and optimised scenarios, stakeholders can now make informed decisions. The decision-making process should be data-driven, considering the potential risks and rewards of each option. It’s important to involve relevant stakeholders from different departments to ensure that the decision aligns with the overall business strategy.
Implementing the chosen solution: Once a decision is made, the next step is to implement the chosen solution. This could involve changes to operational processes, marketing strategies, product offerings, or resource allocation. Effective implementation requires careful planning, clear communication, and coordination across different teams to ensure a smooth transition.
Monitoring and measuring results against targets: After implementation, it’s crucial to continuously monitor the results and measure them against the predefined targets. Tracking relevant metrics and KPIs is necessary to assess the solution’s effectiveness. If the results deviate from the expected outcomes, adjustments may be necessary. Regular monitoring and evaluation ensure that the solution remains effective and helps identify areas for further improvement.
Step 7: Updating the System with the Results of the Decision
The final step in the business analytics process is not an end but a beginning. This phase ensures the ongoing relevance and refinement of the analytics process by incorporating the outcomes of previous decisions.
Feedback Loop for Continuous Improvement: A feedback loop is essential for adapting to changing business landscapes and improving the accuracy of future analyses. By reviewing past decision results on a regular basis, analysts can identify what worked well and what could be improved. This feedback is then used to refine models, processes, and data collection methods, leading to more accurate insights and better decision-making.
Updating Models and Processes Based on Outcomes: As new data becomes available and business environments change, the models and processes used in business analytics must be updated. This ensures that the insights generated remain relevant and accurate. By incorporating the feedback loop, organizations can continuously improve their analytical capabilities and stay ahead of the competition.
Ensuring Data and Insights are Current and Relevant: Data is the lifeblood of business analytics. Keeping data up to date and of high quality is critical for ensuring the accuracy of insights. Outdated or irrelevant data can lead to flawed conclusions and misguided decisions. Regular data audits and updates are essential to ensure that the analysis is based on the most current and reliable information.
Note
Businesses can create a self-improving cycle by closing the loop and continuously updating the system with decision results, which improves their analytical capabilities, drives better decision-making, and ultimately leads to improved business outcomes.
Be part of the tech revolution with Scaler Courses. Gain the expertise to thrive in the ever-evolving field of technology.
Conclusion
The 7-step business analytics process, as outlined above, provides a structured approach for organizations to leverage data for informed decision-making. Businesses can gain valuable insights and drive continuous improvement by defining business needs, exploring and analyzing data, developing predictive models, optimizing solutions, making decisions, measuring outcomes, and constantly updating the system.
It is critical to remember that business analytics is not a linear process but rather an iterative one. The steps are interconnected, and feedback loops ensure that the process adapts and evolves based on new data and changing business needs.
For those looking to excel in the field of business analytics, Scaler’s Data Science course offers a comprehensive curriculum that covers all aspects of the business analytics process. From data collection and analysis to machine learning and model deployment, Scaler provides you with the skills and knowledge you need to make data-driven decisions and drive business results.
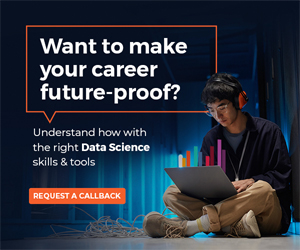
In today’s data-driven world, businesses can gain a competitive advantage, optimize operations, and achieve long-term growth by mastering the 7-step business analytics process and leveraging data science.
FAQs
What is the 5-step business analytics process model?
There is no universally accepted 5-step model, but a common framework includes defining business needs, data exploration, data analysis, predicting outcomes, and optimizing solutions.
What is business analytics a process to?
Business analytics is the process of converting data into actionable insights that drive informed decision-making, increase efficiency, and boost overall business performance.
What are the 5 Ps of analytics?
The 5 Ps of analytics are Purpose (defining the problem), Preparation (data collection and cleaning), Processing (analyzing data), Presentation (communicating findings), and Performance (measuring impact).
What are the 7 techniques of business analysis?
Some common business analysis techniques include SWOT analysis, MOST analysis, PESTLE analysis, MoSCoW prioritization, brainstorming, mind mapping, and root cause analysis.
What is the business analyst process?
The business analyst process involves understanding business needs, gathering and analyzing requirements, designing solutions, and facilitating implementation to improve business processes and achieve organizational goals.