Machine learning, a subset of artificial intelligence, is rapidly transforming industries and changing how we live and work. Its ability to learn from data and make predictions or decisions without explicit programming has opened up a world of possibilities. A report by McKinsey estimates that machine learning could add up to $13 trillion to the global economy by 2030. Machine learning applications are becoming more common in our daily lives, from personalized recommendations on streaming platforms that keep us engaged to self-driving cars that navigate our roads with greater precision.
Machine learning’s widespread adoption stems from its ability to extract valuable insights from massive amounts of data, automate complex tasks, and improve decision-making processes. In healthcare, machine learning algorithms are aiding in disease diagnosis with accuracy rates surpassing 90% in some cases. In finance, it is used for fraud detection, saving businesses billions of dollars each year. In marketing, it powers personalized recommendations, leading to a significant increase in customer engagement and sales.
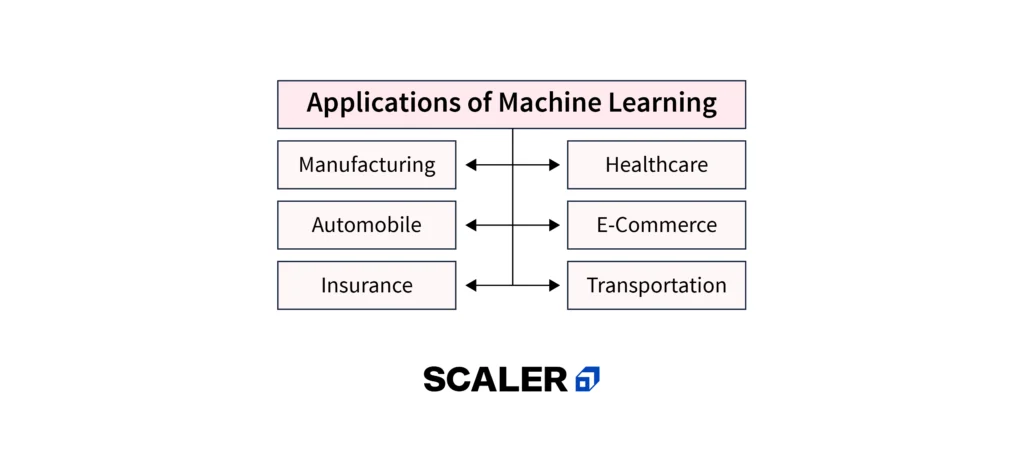
If you’re intrigued by the power of machine learning and eager to delve into this exciting field, Scaler’s Machine Learning Course offers a comprehensive learning path. You can gain the skills and knowledge required to thrive in the world of machine learning by participating in expert-led instruction, hands-on projects, and career guidance.
Let us look at some of the most popular applications of machine learning:
1. Image Recognition
Image recognition, a cornerstone of machine learning, empowers computers to interpret and understand visual information from the world around us. This technology has moved beyond the realm of science fiction to become an essential part of our daily lives. At its core, image recognition involves training algorithms to analyze patterns, textures, and shapes within images, enabling them to identify objects, scenes, and even emotions.
Key Applications of Image Recognition
- Facial Recognition: This application has become ubiquitous, from unlocking our smartphones to enhancing security measures in airports. Facial recognition algorithms use facial features to identify individuals, providing convenience and security in a variety of settings.
- Object Detection: This technology goes beyond identifying the presence of an object; it pinpoints the location of objects within an image or video. Object detection is essential for applications such as self-driving cars, surveillance systems, and augmented reality experiences.
- Image Classification: Image classification categorizes images into predefined classes or labels. This lays the groundwork for applications such as content moderation on social media platforms, image organization in photo libraries, and even medical diagnosis, where algorithms can detect anomalies in scans.
2. Speech Recognition
Speech recognition, also called automatic speech recognition (ASR) or speech-to-text, is a multifaceted technology that allows machines to interpret and transcribe spoken language into text. It begins with converting raw audio signals into a digital format, which is then processed to extract relevant features like phonemes (basic units of sound) and formants (resonant frequencies of the vocal tract). Acoustic models, trained on vast datasets of labelled speech data, are employed to recognize patterns and relationships between sounds and words. Furthermore, pronunciation modeling assists the system in understanding variations in pronunciation and accents, whereas language models analyze the context and grammar of speech to predict the most likely words and phrases, thereby improving accuracy.
Key Applications of Speech Recognition
- Virtual Assistants: Speech recognition powers virtual assistants like Siri, Alexa, and Google Assistant, allowing users to interact with their devices using natural language.
- Transcription Services: Speech recognition is transforming the way we transcribe audio and video content, making it faster, more efficient, and accessible. It is used in a variety of fields, including journalism and legal proceedings.
- Language Translation: Real-time speech translation is breaking down language barriers and facilitating communication across cultures. This technology is invaluable for travellers, businesses, and individuals seeking to connect with people from different linguistic backgrounds.
3. Traffic Prediction
Machine learning is transforming traffic prediction by analyzing massive amounts of data from a variety of sources, such as GPS devices, sensors, historical traffic patterns, and even social media feeds. Sophisticated algorithms can identify patterns, correlations, and trends within this data to make accurate predictions about future traffic conditions.
These algorithms consider a variety of factors, including time of day, weather conditions, road construction, accidents, and special events. By processing and analyzing this data in real-time, machine learning models can anticipate congestion, predict travel times, and suggest alternative routes, ultimately enhancing the efficiency and safety of transportation systems.
Key Applications of Machine Learning in Traffic Prediction
- Real-time Navigation Systems: Apps like Google Maps and Waze rely heavily on machine learning to provide users with accurate, up-to-the-minute traffic information. By continuously analyzing data from millions of users, these apps can dynamically adjust routes and recommend the shortest paths to avoid congestion.
- Traffic Management and Control: Traffic management authorities utilize machine learning to optimize traffic flow, manage congestion, and respond to incidents more effectively. Predictive models can predict traffic bottlenecks and enable proactive measures such as adjusting traffic signal timings or assigning traffic officers to specific areas.
4. Product Recommendations
Product recommendation systems powered by machine learning are changing the way we discover and interact with products and content online. These sophisticated systems analyze user behaviour, preferences, and historical data to tailor suggestions to individual tastes. These systems use techniques such as collaborative filtering (which identifies users with similar preferences), content-based filtering (which analyzes item attributes), and hybrid approaches (which combine both methods) to deliver personalized recommendations that improve user experiences and drive engagement.
Key Applications of Product Recommendations:
- E-commerce Platforms: Online retailers like Amazon and Alibaba harness recommendation engines to boost sales and elevate customer satisfaction. Personalized product recommendations encourage users to try new items and make more purchases.
- Streaming Services: Platforms like Netflix and Spotify employ recommendation algorithms to curate movies, TV shows, or music based on users’ viewing or listening history. This keeps users interested and encourages them to explore new content.
- Personalized Marketing: Recommendation systems are also used in marketing campaigns to deliver targeted advertisements and promotions to individual users, increasing the chances of conversion.
5. Self-Driving Cars
Self-driving cars, also known as autonomous vehicles (AVs), are a game-changing application of machine learning that promises to transform transportation. These vehicles leverage an array of sensors, including cameras, radar, lidar, and ultrasonic sensors, to perceive their surroundings. Machine learning algorithms process this sensory data to make real-time decisions about steering, acceleration, braking, and navigation, enabling the vehicle to operate without human intervention.
Key Applications of Machine Learning in Self-Driving Cars:
- Safety Features: Machine learning plays a crucial role in enhancing vehicle safety. Algorithms can detect lane markings, identify pedestrians and obstacles, and anticipate potential collisions, allowing the vehicle to respond quickly and autonomously to avoid accidents. Features like adaptive cruise control, automatic emergency braking, and lane-keeping assist are all powered by machine learning, significantly reducing the risk of human error on the road.
- Fully Autonomous Vehicles: The ultimate goal of self-driving technology is to achieve full autonomy, where vehicles can navigate and operate in complex environments without any human input. Machine learning is at the heart of this effort, constantly learning from data and honing its decision-making abilities to handle a variety of traffic scenarios, weather conditions, and unexpected events.
While fully autonomous vehicles are still in development, recent years have seen significant progress. Companies like Waymo, Tesla, and Cruise are actively testing and deploying self-driving vehicles in controlled environments, with the promise of a future where transportation is safer, more efficient, and accessible to all.
Discover Scaler’s Machine Learning Course and elevate your tech career today!
6. Email Spam and Malware Filtering
Machine learning is transforming cybersecurity by strengthening our defences against the ever-increasing threat of spam, malware, and phishing attacks. Traditional rule-based filters often struggle to keep up with the evolving tactics of cybercriminals, but machine learning algorithms offer a dynamic and adaptive solution.
These algorithms are trained on massive datasets of emails, learning to distinguish between legitimate messages and malicious content. They examine a variety of features, including email headers, content, sender reputation, and behavioural patterns, to detect subtle hints that indicate spam or malware. By continuously learning and adapting, these algorithms become increasingly accurate at detecting and filtering out unwanted and harmful emails.
Key Applications of Machine Learning in Email Security:
- Spam Detection: Machine learning algorithms can effectively detect and filter spam emails, reducing clutter in your inbox and protecting you from unwanted solicitations and scams.
- Malware and Phishing Prevention: Machine learning plays a crucial role in identifying and blocking emails that contain malware or phishing attempts. These algorithms, by analyzing suspicious links, attachments, and content, can keep you safe from cyber attacks.
The use of machine learning in email security is not only enhancing the safety and security of our digital communications but also improving the overall user experience. With improved spam filtering and malware protection, we can confidently navigate our inboxes and focus on the messages that are truly important.
7. Virtual Personal Assistants
AI-driven virtual personal assistants (VPAs) have become indispensable companions in our increasingly connected lives. These intelligent agents, powered by sophisticated machine learning algorithms, are intended to understand and respond to natural language commands, making them more accessible and user-friendly. They’ve evolved from simple voice-activated tools to sophisticated assistants capable of managing complex tasks and providing personalized experiences.
Key Applications of Virtual Personal Assistants:
- Task Management: VPAs excel at managing our schedules, setting reminders, creating to-do lists, and even booking appointments. They can work seamlessly with our calendars and other apps, streamlining our daily tasks.
- Information Retrieval: Whether it is answering questions, providing weather updates, or locating the nearest restaurant, VPAs can quickly retrieve information from the internet and deliver it in a concise and digestible format.
- Personalization Features: VPAs go beyond simple tasks by learning our preferences and tailoring their responses accordingly. They can recommend music based on our listening habits, suggest movies we might like, and even control smart home devices based on our routines.
8. Online Fraud Detection
Online fraud detection uses machine learning algorithms to analyze massive amounts of transaction data, identifying patterns indicative of fraudulent activity and sending out alerts for further investigation. Utilizing techniques like anomaly detection (flagging unusual patterns), rule-based systems (using predefined rules), and behavioural analytics (analyzing user behaviour over time), these algorithms are becoming increasingly adept at identifying and preventing fraudulent activities.
Key Applications of Machine Learning in Online Fraud Detection:
- Financial Transaction Monitoring: Banks and financial institutions use machine learning algorithms to track transactions in real-time, detecting suspicious activity and preventing fraudulent transactions before they cause financial harm.
- Identity Theft Prevention: By analyzing user behaviour and transaction patterns, machine learning can detect instances of identity theft, such as unauthorized account access or unusual spending patterns.
9. Stock Market Trading
Machine learning is transforming the stock market by providing traders and investors with data-driven insights and automated decision-making tools. By analyzing vast amounts of historical and real-time financial data, machine learning models can identify patterns, trends, and correlations that might not be apparent to human analysts.
Key Applications of Machine Learning in Stock Market Trading:
- Predictive Analytics: Machine learning algorithms can forecast stock prices, market trends, and volatility with impressive accuracy. These predictions are based on a wide range of factors, including historical price data, company financials, economic indicators, news sentiment, and even social media trends. By leveraging these insights, traders can make more informed decisions about when to buy, sell, or hold stocks.
- Automated Trading Systems: Machine learning algorithms can be programmed to execute trades automatically based on predefined criteria or real-time market conditions. These algorithmic trading systems can react to market fluctuations at breakneck speed, potentially generating profits without human intervention.
10. Medical Diagnosis
Machine learning is changing the face of medical diagnosis, providing healthcare professionals with powerful tools to improve accuracy, efficiency, and patient results. By analyzing vast amounts of medical data, including patient records, lab results, imaging scans, and genetic information, machine learning algorithms can identify patterns, correlations, and subtle anomalies that may not be readily apparent to human clinicians.
Key Applications of Machine Learning in Medical Diagnosis:
- Diagnostic Imaging: Machine learning algorithms excel at analyzing medical images, such as X-rays, CT scans, and MRIs. They can detect tumours, fractures, and other abnormalities with incredible precision, often outperforming human radiologists in certain tasks. This not only accelerates diagnosis but also reduces the risk of human error.
- Personalized Treatment Plans: Machine learning can analyze a patient’s unique genetic profile, medical history, and lifestyle factors to develop personalized treatment plans. This tailored approach ensures that patients receive the most effective therapies while minimizing negative side effects.
- Predictive Healthcare Analytics: By analyzing patient data and identifying risk factors, machine learning models can predict the likelihood of developing certain diseases or conditions. This allows for early intervention and preventive measures, which could save lives and improve overall health outcomes.
The application of machine learning to medical diagnosis is still in its early stages, but the potential benefits are enormous. From faster and more accurate diagnoses to personalized treatment plans and predictive analytics, machine learning is poised to transform healthcare and improve patient care.
11. Automatic Language Translation
Automatic language translation, a marvel of artificial intelligence, is transforming the way we communicate across linguistic boundaries. This technology, based on natural language processing (NLP), allows computers to understand, interpret, and generate human language, allowing for seamless communication between individuals and organizations that speak different languages.
NLP algorithms analyze the grammatical structure, semantics, and context of text or speech, allowing them to accurately translate it into another language. These algorithms are trained on massive datasets of bilingual or multilingual text to learn the nuances of language and produce accurate and fluent translations.
Key Applications of Automatic Language Translation:
- Real-time Translation Services: Platforms like Google Translate and Microsoft Translator offer real-time translation capabilities, enabling users to translate text, documents, and even spoken conversations on the fly. This has transformed communication for travellers, businesses, and individuals looking to connect with people of various linguistic backgrounds.
- Multilingual Communication Tools: Automatic language translation is built into a variety of communication tools, including chatbots, email clients, and social media platforms, allowing users to communicate seamlessly in multiple languages.
Unlock the Power of Machine Learning with SCALER
If you’re fascinated by the potential of machine learning and its applications, delve deeper into this transformative field with Scaler’s Machine Learning Course. This comprehensive program covers everything from the fundamentals of machine learning algorithms to cutting-edge applications such as natural language processing and computer vision. Through expert instruction, hands-on projects, and mentorship, you’ll gain the skills and knowledge to build your own machine-learning models and contribute to this exciting field.
Explore the world of machine learning with Scaler and start your path to a rewarding career in this dynamic and rapidly growing field.
Conclusion
Machine learning, a transformative force across industries, is reshaping the way we interact with technology and the world around us. Machine learning has an undeniable impact, from the subtle algorithms that curate our social media feeds to the game-changing potential of self-driving cars. Its applications span diverse domains, enhancing healthcare diagnostics, revolutionizing financial markets, empowering personalized experiences, and fortifying cybersecurity defences. As this technology continues to advance, we can anticipate even more sophisticated applications, such as more accurate medical diagnoses, smarter cities, and enhanced human-computer interactions.
The future of machine learning is brimming with possibilities. As algorithms improve, data sets grow, and computational power grows, we can expect machine learning to tackle even more complex problems, pushing the boundaries of innovation and transforming our lives in ways we can only imagine.
FAQs
What are some common applications of machine learning?
Machine learning has numerous applications, including image recognition for facial identification and object detection, speech recognition for virtual assistants and transcription, traffic prediction for navigation systems, e-commerce product recommendations, self-driving cars, email spam filtering, fraud detection, stock market trading, and medical diagnosis.
How does machine learning improve image recognition?
Machine learning algorithms analyze patterns, textures, and shapes within images to accurately identify objects, scenes, and even emotions, often surpassing human capabilities. This enables applications like facial recognition, object detection, and image classification.
What role does machine learning play in self-driving cars?
Machine learning is the backbone of self-driving cars, processing data from sensors to make real-time decisions about navigation, obstacle avoidance, and safe driving manoeuvres, ultimately aiming for fully autonomous vehicles.
Can machine learning accurately predict stock market trends?
While machine learning models can analyze financial data to identify patterns and trends, stock market prediction remains complex due to market volatility and unpredictable events. However, machine learning can provide valuable insights to aid in informed decision-making.
How does machine learning help in medical diagnosis?
Machine learning analyzes medical images and patient data to assist in diagnosing diseases, identifying anomalies, and developing personalized treatment plans, potentially improving accuracy and efficiency in healthcare.
What is the impact of machine learning on fraud detection?
Machine learning algorithms analyze transaction data to detect patterns indicative of fraudulent activity, helping prevent financial losses and identity theft in the digital landscape.
Are there any limitations to machine learning applications?
Yes, machine learning has limitations. It requires large datasets for training, can be biased based on the data it learns from, and may struggle with interpreting complex or nuanced situations that require human judgment and expertise.