A comprehensive data analyst course syllabus is your roadmap to mastering this in-demand skill set. It gives you the skills and resources you need to gather, purify, examine, and present data in order to find patterns, trends, and connections that could completely change an industry.
Unlock your potential in the data revolution with Scaler’s Data Science course. Master the skills to transform unstructured data into actionable insights and drive strategic decisions. Enroll now and start your journey towards becoming a data expert!
This guide will take you through the key components of a well-rounded data analyst course syllabus in 2025, highlighting the essential topics and skills you need to become a successful data analyst. This syllabus break-down will give you a clear route to data mastery, regardless of your level of experience—from novices looking to launch their careers to seasoned professionals looking to advance your skills.
What is Data Analytics?
Data analytics is the process of examining large datasets to uncover patterns, trends, and insights that can be used to make informed decisions. It involves collecting, cleaning, analyzing, and interpreting data to extract meaningful information. Data analysts use various tools and techniques to analyze data, including statistical analysis, data mining, and machine learning.
Data analytics has become an essential tool for businesses in today’s data-driven world. It enables organizations to gain a deeper understanding of their customers, optimize their operations, and identify new opportunities for growth.
Data Analyst Course Syllabus
A well-designed course on data analytics gives you the abilities and information required to glean insightful conclusions from data and facilitate well-informed decision-making. Here’s a comprehensive syllabus outline for 2025, covering both foundational and advanced topics:
1. Business Analytics Using Excel
This course provides a solid foundation in business analytics using Excel, a widely used tool for data analysis. You’ll learn how to clean, prepare, and analyze data using Excel’s functions and tools, as well as create effective data visualizations to communicate insights.
Key Educational Outcomes:
- Develop proficiency in using Excel for data analysis and visualization.
- Learn to apply statistical and financial functions to analyze data.
- Create effective data visualizations using Excel’s charting tools.
- Understand basic business analytics concepts and metrics.
Course Outline:
- Data cleaning and preparation
- Descriptive statistics
- Data visualization techniques
- Financial analysis
- Case studies in business analytics
2. SQL Fundamentals
SQL is a powerful language for interacting with databases and extracting valuable information. This course will teach you the fundamentals of SQL syntax, database design, and query writing.
Key Learning Outcomes:
- Master the fundamentals of SQL syntax and database management.
- Query databases efficiently to extract relevant data.
- Understand database design concepts and normalization.
- Work with different database management systems (DBMS).
Course Structure:
- Introduction to SQL
- Data types and operators
- Database design
- SQL queries (SELECT, INSERT, UPDATE, DELETE)
- Advanced SQL concepts (joins, subqueries, window functions)
3. Programming and Data Analytics with Python
Python is a versatile language widely used for data analysis. This course will equip you with the skills to use Python for data manipulation, visualization, and statistical analysis.
Key Educational Goals:
- Learn the Python programming language for data analysis.
- Utilize Python libraries like NumPy, Pandas, and Matplotlib for data manipulation and visualization.
- Apply statistical and machine learning techniques using Python.
- Develop data-driven applications and solutions.
Course Modules:
- Python programming fundamentals
- Data manipulation and cleaning with Pandas
- Statistical analysis with Python
- Machine learning concepts and algorithms
- Data visualization with Matplotlib and Seaborn
4. Data Science with R Programming
R is another popular language for data analysis, offering a rich ecosystem of packages for various tasks. This course will teach you how to use R for data science and analysis.
Key Learning Outcomes:
- Master the R programming language for data analysis and visualization.
- Utilize R packages like dplyr, ggplot2, and caret for data manipulation and modeling.
- Apply statistical and machine learning techniques using R.
- Develop data-driven solutions and models.
Course Framework:
- R programming fundamentals
- Data manipulation and cleaning with dplyr
- Statistical analysis with R
- Machine learning concepts and algorithms
- Data visualization with ggplot2
5. Tableau for Data Visualization
Tableau is a powerful tool for creating interactive and visually appealing data visualizations. This course will teach you how to use Tableau to effectively communicate data insights.
Key Learning Goals:
- Create interactive and visually appealing data visualizations using Tableau.
- Understand Tableau’s interface and features.
- Build dashboards and reports to communicate data insights effectively.
- Customize visualizations to meet specific business requirements.
Program Outline:
- Tableau basics and interface
- Data sources and connections
- Data modeling and transformations
- Creating visualizations and dashboards
- Sharing and collaborating on reports
6. Power BI Training
Power BI is another popular tool for data visualization and reporting. This course will teach you how to use Power BI to create interactive dashboards and analyze data.
Key Educational Outcomes:
- Learn to use Power BI for data visualization and reporting.
- Connect to various data sources and create interactive dashboards.
- Use Power BI’s features for data modeling and analysis.
- Share and collaborate on Power BI reports.
Course Curriculum:
- Power BI basics and interface
- Data sources and connections
- Data modeling and transformations
- Creating visualizations and dashboards
- Sharing and collaborating on reports
7. Capstone Project: Data Analyst
A capstone project is a necessary component of the perfect data analyst curriculum. Students can demonstrate their knowledge to potential employers by applying their talents to address a real-world problem through a capstone project. The project would show that the student is proficient in problem-solving, data analysis, and visualisation.
How to Get Started as a Data Analyst?
Here are some steps to consider when starting your journey as a data analyst:
- Gain a Solid Foundation: Acquire the necessary skills by completing a data analytics course or earning a relevant degree.
- Build a Strong Portfolio: Demonstrate your skills by working on personal projects or contributing to open-source data analysis initiatives.
- Network with Industry Professionals: Connect with other data analysts, data scientists, and industry experts to learn from their experiences and build relationships.
- Practice Data Analysis Techniques: Regularly practice data analysis techniques using real-world datasets to hone your skills.
- Stay Updated with Industry Trends: Keep up with the latest developments in data analytics and technology to remain competitive.
- Learn Popular Tools: Become proficient in commonly used data analysis tools like Python, R, SQL, Excel, Tableau, and Power BI.
Take the first step towards becoming a data analytics expert with Scaler’s Data Science course. Master essential tools like Python, R, SQL, and Tableau to transform data into actionable insights. Enroll now and elevate your career!
Software and Tools Used in Data Analyst
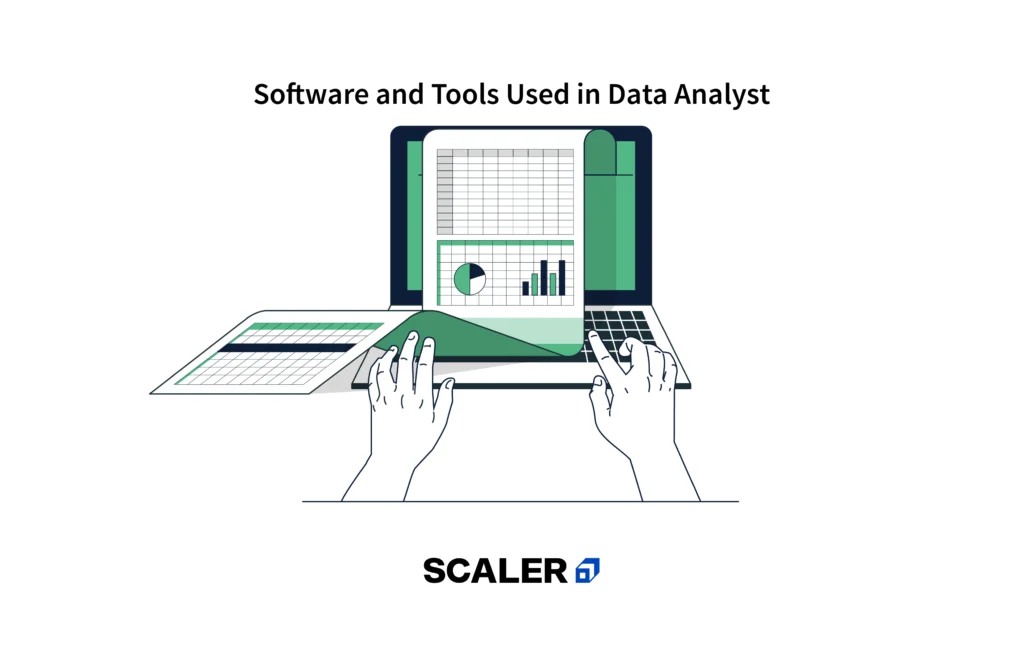
A data analyst can extract valuable insights from data by using a variety of powerful software and tools that are part of their toolkit. These tools are not just theoretical concepts but essential instruments used daily in real-world scenarios. Let’s explore some of the most common ones:
1. Microsoft Excel
Excel, the widely used spreadsheet program, is a data analyst’s best friend. Its user-friendly interface and built-in functions allow for data organization, basic calculations, and simple visualizations. You can learn how to use Excel’s features to clean and prepare data, do calculations with formulas and functions, make pivot tables for data summarization, and produce simple charts and graphs for data visualization by enrolling in a data analytics course.
- Practical Applications: Excel is widely used for tasks like budgeting, financial analysis, inventory management, and creating reports. In a course setting, you might use Excel to clean a dataset, analyze sales figures, or create a chart to visualize customer demographics.
2. Python
Python, with its simplicity and readability, is a powerful language for data analysis. In a data analytics course, you’ll dive into Python’s rich ecosystem of libraries, including:
- Pandas: Used for data manipulation and analysis, allowing you to easily clean, transform, and aggregate data.
- NumPy: Provides support for numerical operations on arrays and matrices, making it essential for scientific computing and data analysis.
- Matplotlib and Seaborn: Used for creating a wide range of static, animated, and interactive visualizations.
- Practical Applications: Python is used in various industries for tasks like data cleaning, web scraping, statistical analysis, building machine learning models, and creating data-driven web applications. Python can be used in a classroom to create a basic recommendation system, forecast sales trends, and analyze customer data.
3. R Programming
R is a language created especially for graphic design and statistical computing. It offers a comprehensive set of tools for data analysis, visualization, and statistical modeling.
- Practical Applications: R is used extensively for tasks like data exploration, hypothesis testing, regression analysis, and producing plots of publication quality in academia, research, and industries such as finance, healthcare, and marketing. In a course setting, you might use R to perform statistical analysis on survey data or build a predictive model for customer churn.
4. SQL (Structured Query Language)
The language used by databases to store, retrieve, and manipulate structured data is called SQL. In a data analytics course, you’ll learn how to write SQL queries to extract data from databases, filter results, aggregate information, and join tables to combine data from multiple sources.
- Practical Applications: SQL is used in virtually every industry that relies on data, from banking and finance to healthcare and e-commerce. In a course setting, you might use SQL to retrieve customer data from a database, analyze sales figures, or track website traffic patterns.
5. Tableau & Power BI
These powerful data visualization tools allow you to create interactive dashboards and reports that transform complex data into easy-to-understand visuals. In a data analytics course, you’ll learn how to connect these tools to various data sources, design visually appealing dashboards, and create interactive visualizations that tell a story with data.
- Practical Applications: Tableau and Power BI are widely used in business intelligence and analytics for tasks like monitoring key performance indicators (KPIs), tracking sales performance, analyzing customer behaviour, and reporting financial results. In a course setting, you might use Tableau or Power BI to create a dashboard that tracks website traffic or visualizes customer demographics.
Conclusion
A comprehensive syllabus for a data analytics course serves as your road map for utilizing data’s potential. From foundational concepts in statistics and programming to advanced techniques like machine learning and data visualization, mastering these skills equips you with the tools to transform raw data into actionable insights that drive business growth.
The curriculum we have laid out includes important topics like statistical analysis, data cleaning, database management with SQL, Python and R programming languages, and robust visualization tools like Tableau and Power BI. By gaining proficiency in these areas, you’ll be well-prepared to tackle real-world data challenges, communicate findings effectively, and make a significant impact in your chosen field.
Accelerate Your Data Analytics Journey with SCALER
If you’re looking for a comprehensive and career-focused data analytics program, Scaler’s Data Science and Machine Learning Course is a great option. Along with covering the essential topics covered in the syllabus, it also provides you with real-world projects, career guidance, and one-on-one mentoring to help you get started in the data analytics field.
With Scaler, you can develop the useful abilities and self-assurance required to take on challenging data problems, create predictive models, and effectively share insights—all while getting professional advice and assistance from industry experts.
FAQs
What prerequisites are required for a data analytics course?
A basic understanding of statistics and mathematics is required for most data analytics courses. Some may also require prior knowledge of programming languages like Python or R. However, many beginner-level courses cater to individuals with no prior experience.
How long does it take to complete a data analytics course?
Depending on the depth and intensity of the program, the duration varies. Online courses can range from a few weeks to several months, while bootcamps typically last 3-6 months. Master’s programs usually take 1-2 years.
What job roles can I expect after completing the course?
After completing a data analytics course, you can pursue roles like Data Analyst, Business Analyst, Marketing Analyst, or Operations Analyst. With further specialization, you can also transition into roles like Data Scientist or Machine Learning Engineer.
Are there any certifications included in the course?
Some courses offer certifications upon completion, either from the course provider itself or through partnerships with external organizations. These certifications can validate your skills and enhance your resume.
What kind of projects will be part of the course curriculum?
Data analytics courses typically include hands-on projects that simulate real-world scenarios. These projects may involve analyzing customer data, predicting sales trends, or creating interactive dashboards to visualize key business metrics.