In today’s data-driven world, data engineers and scientists play an important role in building hi-tech tomorrow. While both professionals work with a huge amount of data extensively, their duties and skill sets differ greatly. This article provides an in-depth comparison of data engineers versus data scientists, including job descriptions, wages, educational requirements, and more. Understanding the distinctions between these two positions allows one to choose their preferred domain and understand their niche.
What are the Differences Between a Data Engineer and a Data Scientist?
Let us look at the differences between a Data Engineer and a Data Scientist, two critical professions in data-driven decision-making.
Who Is a Data Engineer?
A Data Engineer is a professional who is in charge of planning, building, and maintaining the architecture that enables the collection and storage of massive volumes of data. They specialize in building strong pipelines that provide continuous data flow and analysis accessible.
Who Is a Data Scientist?
A Data Scientist specializes in extracting insights and patterns from data using advanced statistical and machine-learning approaches. They analyze complex datasets to extract actionable insights and contribute to strategic decision-making.
Interested in a career as a data engineer or data scientist? Explore how Scaler’s Data Science Course can equip you with the skills and knowledge to excel in these dynamic fields. Dive into practical learning with industry experts and advance your career in data-driven decision-making. Enroll today and take your first step towards a rewarding future in data science!
Comparison of Data Scientist and Data Engineer: Roles, Responsibilities, and Skills
When comparing data scientists with data engineers, several crucial factors come into play:
Aspect | Data Scientist | Data Engineer |
---|---|---|
Role | They extract insights and develop prediction models. | They construct and manage data infrastructure. |
Responsibilities | They are in charge of data analysis, model development, and data visualization storytelling. | They are responsible for data pipeline building, database administration, and data quality assurance. |
Skills | – Require extensive statistical knowledge – Expertise in machine learning methods – Experience with data visualization tools such as Tableau or Matplotlib. | – Must be skilled in programming languages such as Python and SQL – Have an understanding of tools such as Apache Spark, Hadoop, and ETL (Extract, Transform, Load) procedures. |
Tools and Technologies | Data Scientists use libraries and tools like: TensorFlow, Sci-kit-learn, Jupyter Notebooks, Pandas, Matplotlib, NumPy, Seaborn, Keras, Pytorch, SQL, Tableau and Power BI. | Data Engineers use tools like: Apache Kafka, Apache Airflow, MySQL, PostgreSQL, SAP, Oracle, Cassandra, Redis, Riak, MongoDB, Neo4j, Hive and Sqoop. |
Understanding these distinctions becomes essential for organizations seeking to effectively use the knowledge of both Data Engineers and Data Scientists in their data-driven initiatives.
Data Engineer Vs Data Scientist: Salary, Education, Career Growth, and More
Let us now look into the differences between data engineers and data scientists, focusing on aspects like education, salary, career trajectory, and job outlook.
Comparing Education & Background
Understanding the educational paths and backgrounds is essential for distinguishing between data engineers and data scientists.
Data Engineer-Specific Education
Data engineers often come from a software engineering background and are proficient in programming languages such as Java, Python, SQL, and Scala. Alternatively, they may have degrees in mathematics or statistics, allowing them to use a variety of analytical methodologies to efficiently address business challenges.
A bachelor’s degree in computer science applied mathematics, or information technology is frequently required to qualify for positions in data engineering. Many employers respect data engineering certificates like Google’s Professional Data Engineer and IBM’s Certified Data Engineer. Experience building huge data warehouses capable of running Extract, Transform, and Load (ETL) operations on large datasets is also advantageous.
Data Scientist-Specific Education
Data scientists are frequently tasked with analyzing massive datasets without stated business challenges, which demands a comprehensive knowledge base that includes approaches in big data infrastructures, data mining, machine learning algorithms, and statistics. They often hold graduate degrees in subjects such as statistics, mathematics, or computer science.
Data scientists must be proficient in programming languages such as SQL, Python, R, and Java, as well as knowledgeable about technologies such as Hive, Hadoop, Cassandra, and MongoDB.
Furthermore, data scientists frequently have a better understanding of business domains, as indicated by their backgrounds in econometrics, mathematics, statistics, and operations research. This broader perspective enables them to define analytical developments in terms of business objectives and outcomes.
Common Backgrounds
Both data engineers and data scientists often have computer science degrees, which give a strong basis for their separate responsibilities. However, data scientists frequently augment their education with courses in econometrics, mathematics, statistics, or operations research, which provides them with extra business knowledge. Data engineers, on the other hand, may have an engineering background and prior computer engineering schooling.
Professionals in the data science business come from a variety of disciplines, including physics, biology, meteorology, web development, and database management. Many people come to data science from other professions, enhancing the profession with diverse perspectives and experiences.
Data Scientist vs. Data Engineer Salaries
Let us compare the salaries of data scientists and data engineers based on several factors such as role, seniority, country, and industry.
Aspect | Data Engineer Salary | Data Scientist Salary |
---|---|---|
Entry Level | ~₹6,00,000 – ₹8,00,000 | ~₹7,00,000 – ₹10,00,000 |
Mid Level | ~₹8,00,000 – ₹12,00,000 | ~₹10,00,000 – ₹15,00,000 |
Senior Level | ~₹20,00,000 and more | ~₹15,00,000 and more |
India | ₹8,00,000 | ₹12,88,691 |
United States | $114,564 | $102,988 |
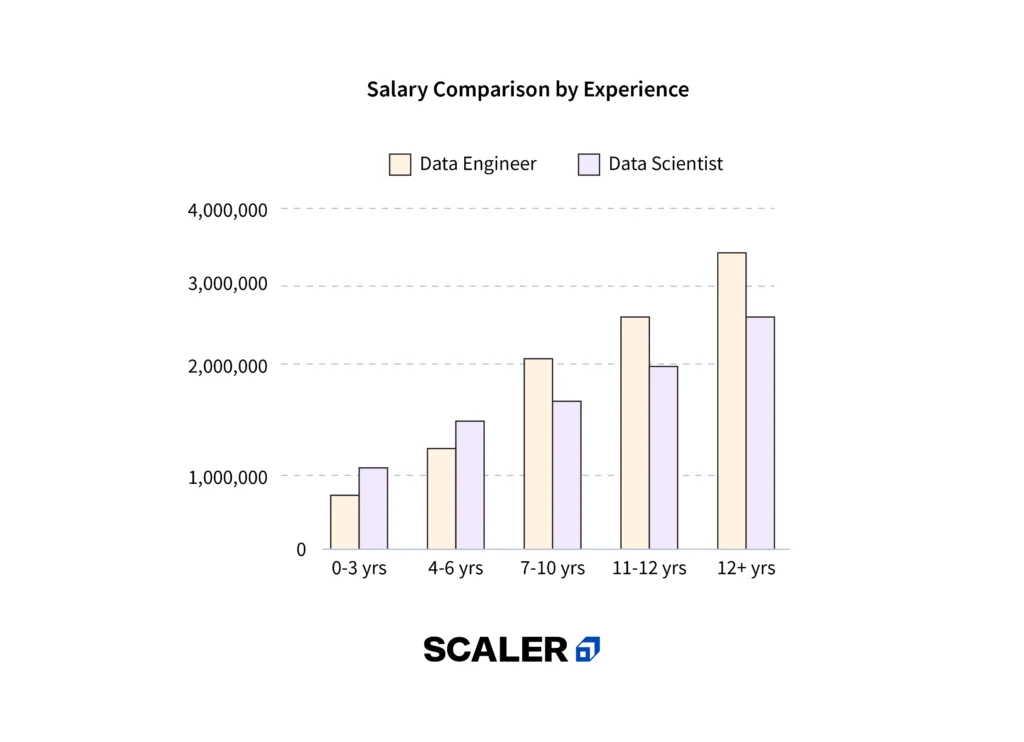
In the above graph, we can see the salary comparison of the data engineer and data scientist based on their years of experience.
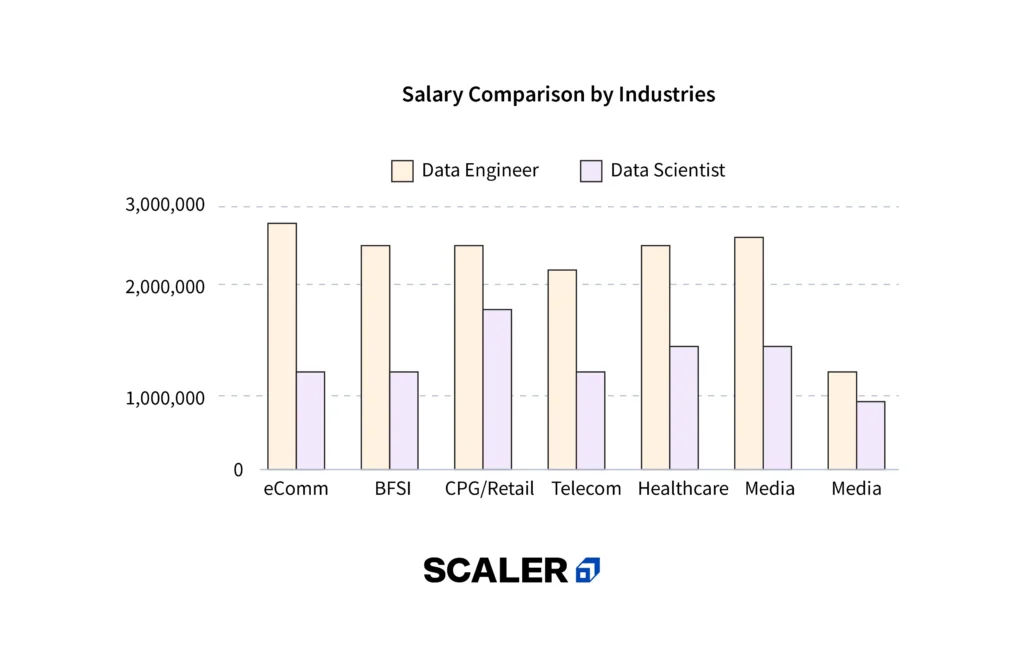
Similarly in the above graph, we can see the difference between the salaries of the data engineer and data scientist based on various industries.
Salary Trend Over The Years
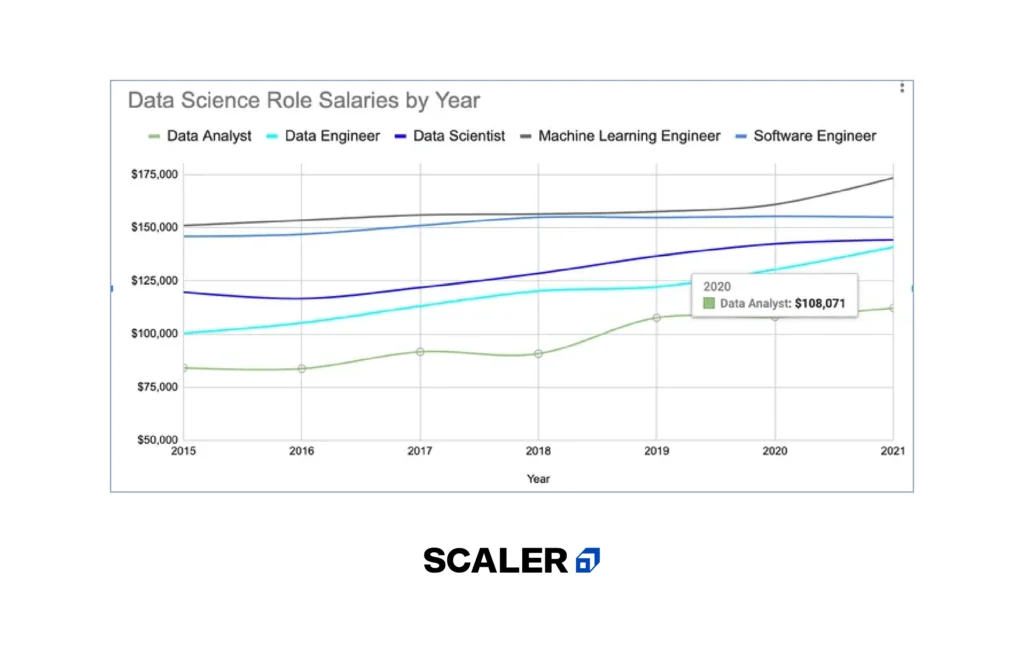
If we look at the graph provided above, we can focus on two lines (data engineer and data scientist), we can see how initially the salary of data engineers used to be lower than data scientists in the years 2015-2020 but in recent years their salary gap has decreased a lot.
Top Companies Hiring
Here are some top companies hiring for data engineers and data scientists across various industries:
Data Engineer
- Amazon
- Microsoft
- Netflix
Data Scientist
- IBM
- Apple
- Airbnb
- Uber
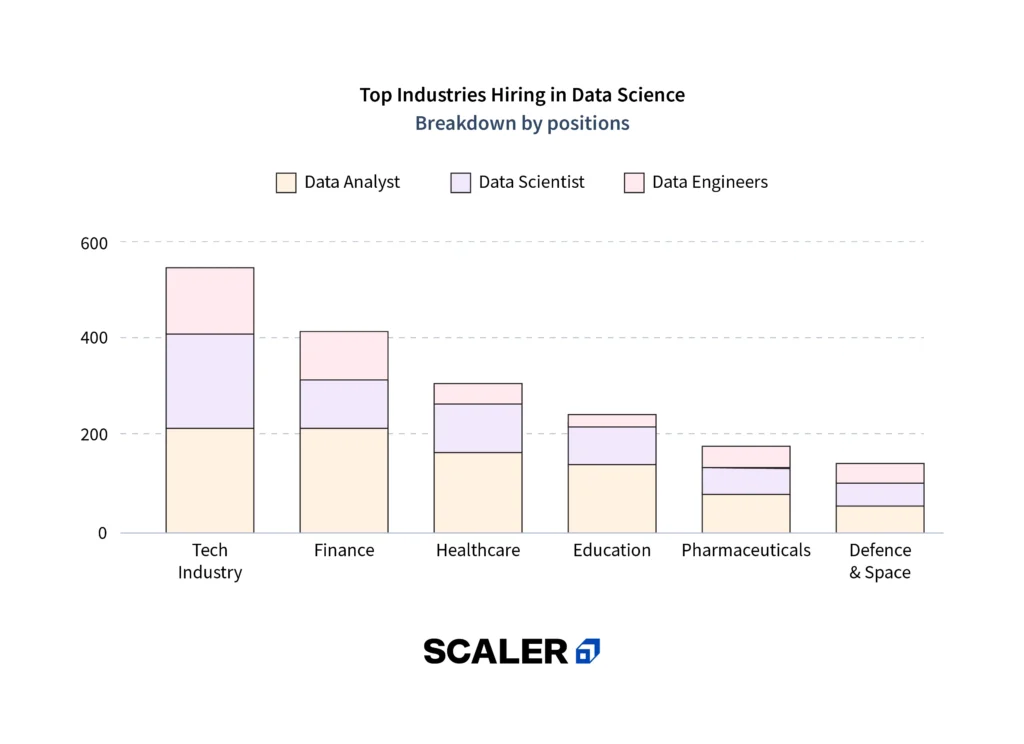
Job Outlook Over the Next 10 Years
The future possibilities for data engineers and scientists are promising.
Data Engineer
The need for qualified data engineers is expected to rise as data-driven decision-making processes become more widely used across businesses. As firms increasingly rely on data to support strategic objectives, there is a greater demand for experts who can design, construct, and maintain effective data infrastructures.
According to industry experts, the rise of cloud computing and the introduction of data lakes as scalable data storage solutions are driving up the need for data engineers. Companies are looking for cost-effective and flexible data management and processing options, which has led to investments in cloud-based solutions.
Data engineers generally begin their careers in software engineering or database administration, where they get experience with important systems and infrastructure. Data engineers may rise to more senior positions, such as data architects or data engineering managers, by using their knowledge of data processing, cloud computing, and ETL techniques.
Data Scientist
Data scientists are in high demand as organizations seek to extract relevant insights from massive datasets to promote innovation and achieve a competitive edge. However, recruiting patterns are shifting, with organizations focusing on establishing diverse data science teams rather than chasing invisible “unicorn” data scientists with extensive skill sets.
While the need for data scientists remains high, employers are prioritizing applicants who possess a combination of technical proficiency, communication skills, creativity, and domain understanding. This trend reflects the changing nature of data science professions, which prioritize cooperation and multidisciplinary problem-solving.
Data scientists’ careers often begin with entry-level employment such as junior data scientists or data analysts, which allow them to refine their technical abilities and work on given projects. Many experts go into data science from other professions, utilizing their knowledge in analytics or statistics to grow into higher-level jobs.
Insights and Projections
Industry estimates present a positive picture for both data engineers and data scientists, with steady demand projected in the coming years. The US Bureau of Labour Statistics predicts a significant number of employment vacancies in both disciplines, highlighting the bright future for data science specialists.
As organisations continue to invest in data-driven strategies and technology, the need for talented people capable of using data to make strategic decisions will remain high. While individual career routes may differ, professionals may take advantage of a wide range of possibilities in the dynamic and ever-changing field of data engineering and data science.
Excited about the future of data science and data engineer? Explore how Scaler’s Data Science Course can equip you with the skills and expertise to thrive in this rapidly growing field. Dive into hands-on learning with industry professionals and advance your career in data-driven decision-making. Enroll now and start your journey towards becoming a sought-after data scientist!
Common Career Paths for Data Engineers and Scientists
Understanding the average professional path might provide insight into potential promotion chances.
Career Path for Data Engineers
Data engineers often start their careers as software engineers or database administrators, gaining foundational skills and experience in data management and software development. With time and expertise, they may advance to roles like senior data engineers, data architects, or data engineering managers, where they lead teams and oversee the design and implementation of complex data systems.
Career Path for Data Scientists
Data scientists typically begin their careers as statisticians, analysts, or junior data scientists, where they develop their skills in data analysis and machine learning. As they gain experience and expertise, they may advance to roles such as senior data scientists, machine learning engineers, or data science managers, where they lead teams and drive the development of advanced analytics and machine learning models.
Can a Data Scientist Become a Data Engineer or Vice Versa?
Yes, it’s entirely possible for a data scientist to transition into a data engineering role, and vice versa, although it may require additional skills and training.
Data Scientist to Data Engineer
A data scientist may transition into a data engineering role by acquiring skills in areas such as database management systems, data warehousing, ETL (extract, transform, load) processes, and software engineering. They may need to learn languages like SQL, Java, Scala, or Python more deeply, focusing on building scalable data pipelines, optimizing data storage and retrieval, and ensuring data quality and reliability. Additional knowledge in cloud computing platforms like AWS, Azure, or Google Cloud can also be beneficial for transitioning into data engineering roles.
Data Engineer to Data Scientist
Similarly, a data engineer interested in becoming a data scientist will be required to improve their abilities in statistics, mathematics, machine learning, and data analysis. They may need to learn computer languages used in data science, such as Python or R, as well as expand their knowledge of advanced analytics, predictive modelling, and experimental design. Deep learning frameworks such as TensorFlow or PyTorch, as well as data exploration and visualization tools such as Jupyter Notebooks, might help with the shift to data science roles.
In both circumstances, acquiring hands-on experience through projects, online courses, boot camps, or formal education programs can assist ease the move. Additionally, networking with individuals in the target sector, seeking mentorship, and harnessing transferable abilities from past employment can help speed up the transition process. While transferring professions might be difficult, the overlap in skills and the growing demand for adaptable people in the data business make such transfers possible with determination and a willingness to learn.
Essential Steps to Start Your Career as a Data Engineer or Data Scientist
Are you interested in data and its ability to generate insights and innovation? Starting a career as a data engineer or data scientist could be your career decision. But where do you start? Here are some key actions to put you on the right track.
First, familiarize yourself with the essentials. Both data engineering and data science necessitate a strong background in mathematics, statistics, and programming. Begin by developing your skills in data-related languages such as Python, R, and SQL.
Next, consider seeking appropriate certifications or courses to improve your expertise and credentials. Certifications like Certified Analytics Professional (CAP) and Google Certified Professional Data Engineer can demonstrate your knowledge in the discipline. Additionally, taking classes from recognized platforms such as Scaler, Coursera, edX, or Udacity can give comprehensive learning experiences targeted to your specific needs.
One prominent choice is the Scaler Academy’s Data Science course, which is aimed to provide determined professionals with industry-relevant abilities. Programs like these might help you get started in the data domain faster by providing hands-on tasks and guidance.
Internships, freelance work, and personal initiatives all provide opportunities to get practical experience. Real-world experience helps you to apply theoretical information, improve your problem-solving skills, and create a portfolio that demonstrates your strengths to potential employers.
Keep up with the latest developments and trends in data engineering and data science. Technology evolves quickly, and staying ahead of the curve might give you an advantage in the job market. Follow industry blogs, attend webinars, and devote time to continual learning.
Finally, make sure your résumé and online profiles showcase your relevant talents, projects, and expertise. Create intriguing cover letters that demonstrate your skills for data and willingness to contribute to the subject.
Following these important instructions will allow you to embark on a productive career as a data engineer or data scientist. With determination, perseverance, and the correct resources, you may transform your passion for statistics into a rewarding career.
Conclusion: Which is Better For You?
Finally, the decision between data engineering and data science is based on your interests, talents, and professional ambitions. If you enjoy interacting with data infrastructure, optimizing data pipelines, and developing scalable systems, data engineering may be a good fit for you. On the other hand, if you enjoy extracting insights from data, creating predictive models, and solving challenging problems, data science could be a good fit.
Consider your strengths and preferences. Are you more interested in coding, software development, and system architecture? Or do you enjoy analyzing data, identifying trends, and explaining the results? Reflecting on these factors can help you make an informed decision.
Finally, there is no one-size-fits-all solution. Both data engineering and data science provide exciting career paths with plenty of possibilities for advancement and creativity. Choose the path that best suits your interests, capitalizes on your strengths, and corresponds with your long-term goals.
FAQs
If my coding skills are weak, should I pursue data engineering or data science?
Both data engineering and data science positions demand coding skills but with distinct emphasis. Data engineering is often more focused on creating and optimizing data pipelines. If you have limited coding skills, data science may be a better fit because it requires more analysis and modeling than infrastructure development does. However, strengthening your coding skills is critical to succeed in either sector.
What are the differences in data scientist and data engineer skills?
Data scientists specialize in analyzing and deriving insights from data. Their skills include proficiency in statistical analysis, machine learning algorithms, programming languages like Python and R, and data visualization tools.
On the other hand, data engineers focus on building and maintaining data infrastructure and pipelines. Their skills include proficiency in programming languages like Python, Java, or Scala, experience with distributed computing frameworks like Apache Hadoop, and Spark, knowledge of database systems, and expertise in data warehousing solutions.
Do data scientists earn more than data engineers?
Salary levels vary depending on experience, region, industry, and company size. Data scientists typically receive slightly higher wages because of the specialized nature of their work and the necessity for superior analytical skills. However, data engineers frequently receive competitive pay, especially those with experience and competence in in-demand technologies.
Is data engineering more challenging than data science?
The difficulty of data engineering versus data science varies according to individual capabilities and preferences. Data engineering includes developing and maintaining an adaptable data infrastructure, which can be difficult due to the complexity of data systems and the requirement for strong programming and database abilities.
Data science, on the other hand, requires in-depth analysis, statistical modelling, and algorithm development, which may pose challenges in data interpretation and problem-solving. Both roles require continuous learning and adaptation to evolving technologies, which may pose challenges in data interpretation and problem-solving. Both roles require continuous learning and adaptation to evolving technologies.
Are data engineers still in demand?
Yes, data engineers are still in great demand as firms rely more on data-driven decision-making and need scalable, efficient data infrastructure. With the exponential growth of data, there is an ongoing demand for people who can design, deploy, and optimise data pipelines to support business operations and analytics.
Is data engineering a promising field for the future?
Yes. With increasing data across businesses and the growing acceptance of cloud computing and big data technologies, data engineering offers significant job opportunities. As organizations attempt to gain actionable insights from large amounts of data, talented data engineers will play a critical role in developing and maintaining the necessary infrastructure.
Is data science considered a safe career choice?
Data science is typically viewed as a secure career path due to its high demand, competitive pay, and area for advancement. As businesses across industries recognize the importance of data-driven decision-making, the demand for skilled data scientists grows. To be competitive in the field, you must stay up to date on emerging technology and constantly improve your skills.