In today’s fast-changing tech world, data science stands out as a highly desirable field of study. With organizations relying more on data for making decisions and staying ahead, the need for skilled data scientists has surged. But is learning data science as hard as people say? This article will explore the factors influencing the perception of data science as a challenging field and uncover the truth behind it. If you’re worried about the learning curve, Scaler’s Data Science Course offers a structured curriculum, expert mentorship, and hands-on projects to make your journey smoother and more enjoyable.
Is Data Science Worth the Hype?
Data science has undeniably gained significant traction in recent years, with a surge in demand for skilled professionals. However, it’s essential to assess whether the hype surrounding data science aligns with the reality of the field.
High Demand and Growing Opportunities:
The field of data science is experiencing rapid growth, driven by the increasing value of data-driven insights. Job opportunities for data scientists are abundant across various industries, from technology and finance to healthcare and marketing. The U.S. Bureau of Labor Statistics predicts a 36% job growth for data scientists between 2021 and 2031, much faster than the average for all occupations.
Competitive Salaries:
Data scientists command competitive salaries, reflecting the high demand for their skills and expertise. According to Glassdoor, the average base salary for a data scientist in India is ₹12,60,468 per year. Salaries can vary depending on factors such as experience, location, company size, and specific skills.
Intellectual Stimulation and Problem-Solving:
Data science offers a highly intellectually stimulating career, involving complex problem-solving, data analysis, and model development. The ability to extract meaningful insights from data and drive informed decision-making is both challenging and rewarding.
Continuous Learning and Development:
The field of data science is constantly evolving, requiring professionals to stay updated with the latest trends and technologies. This continuous learning can be intellectually stimulating and foster a growth mindset.
Challenges and Considerations:
While data science offers many benefits, it’s important to acknowledge the challenges involved. Acquiring the necessary skills and knowledge can be demanding, and the field requires a strong foundation in technical skills, problem-solving, and critical thinking. Additionally, the competitive nature of the field means staying updated with the latest trends and technologies is essential.
Despite the challenges, data science presents a promising career path with high demand, competitive salaries, and intellectual stimulation. If you possess a passion for data, enjoy problem-solving, and are willing to invest in continuous learning, data science can be a rewarding and fulfilling career choice.
Why Data Science is Considered Hard to Learn?
While the rewards of a career in data science may be alluring, it is undeniable that the field is often considered challenging to grasp. Let’s explore some of the key reasons behind this perception:
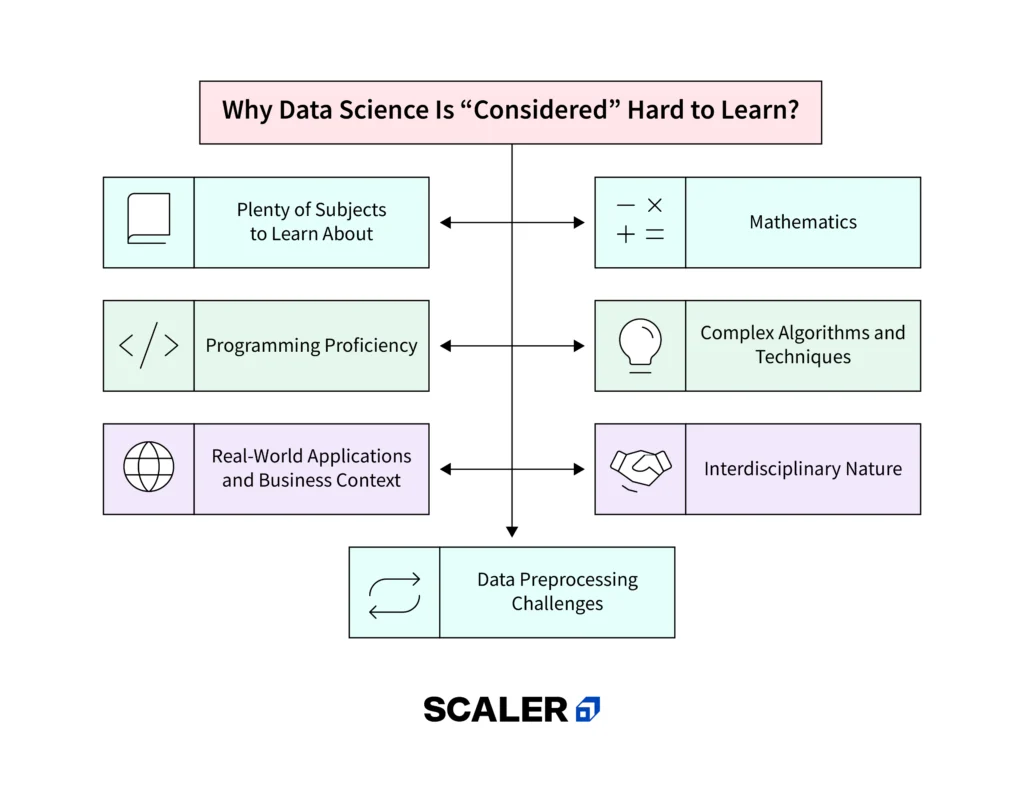
1. Plenty of Subjects to Learn about
Data science isn’t limited to just one or two subjects; it’s a mix of math, stats, coding, and specific industry knowledge. This wide range means you’ll need to learn a lot, from tricky math ideas to becoming skilled in programming languages and understanding the specific needs of different industries.
There’s a lot to learn, but that’s what makes data science so exciting! It keeps your brain sharp and opens doors to all sorts of cool career paths.
2. Mathematics
Mathematics is really important in data science because it’s the base for making models and analyzing data. Being good at things like algebra, calculus, and probability is key for creating stats models, making algorithms, and using data to make decisions. If you’re not great at math, this part of data science might be tough and take a while to get the hang of.
3. Programming Proficiency
Being really good at programming languages like Python or R is super important for data scientists. These languages help you work with data, make graphs, and use machine learning tools. But for people just starting out with no coding experience, learning to code can feel pretty overwhelming.
4. Complex Algorithms and Techniques
Data science is like cracking a complex code. We use various algorithms, from familiar techniques like predicting house prices to cutting-edge deep learning models, to unlock hidden stories within data. Each serves a specific purpose, and mastering them requires dedication and practice. But it’s not just memorizing formulas – it’s about understanding how they work and choosing the right tool for the job.
5. Real-World Applications and Business Context
Data science isn’t just about fancy formulas. It’s about using data to solve real-world problems and drive business success. The key is connecting the dots between complex theories and practical applications. This can feel tricky at first, but with hands-on experience and real-world datasets, you’ll bridge that gap in no time.
Here’s what makes the practical side of data science so powerful:
- Actionable Insights: Data science helps you see beyond the surface, uncovering valuable insights that inform smarter decision-making.
- Real-World Solutions: These insights translate into tangible solutions for businesses, from optimizing marketing campaigns to predicting customer behavior.
- Business Growth: By utilizing data effectively, businesses can gain a competitive edge, improve efficiency, and ultimately, drive growth.
6. Interdisciplinary Nature
Data science isn’t just about crunching numbers. It’s a powerful blend of skills from different areas, like computer science, statistics, and even the specific field you’re working in (like healthcare or finance). This "all-hands-on-deck"
approach means data scientists need to be team players. They collaborate with experts from various backgrounds, communicate clearly, and translate real-world problems into solutions powered by data.
While mastering the technical side of things is crucial, honing these "soft skills"
can be tricky for learners. But don’t worry, the rewards are worth the effort!
7. Data Preprocessing Challenges
Imagine you have a box full of Legos, but some pieces are missing and others are all mixed up. That’s kind of what data scientists deal with before they can analyze information. We call this messy data "raw data,"
and it needs some cleaning up before it’s useful. Here are some of the challenges data scientists face when getting their data ready:
- Missing Pieces: Sometimes, data points are like those missing Legos. We need to figure out how to fill in the gaps or work around them.
- Oddballs Out There: Just like a rogue Lego that doesn’t belong, there can be data points that seem way off. We need to decide if they’re mistakes or important clues.
- Data Doesn’t Always Fit: Think of data sets as different Lego kits – sometimes they don’t connect easily. We gotta merge them smoothly for analysis.
Cleaning up the data takes some time, but it’s worth it! Data science is a growing field where businesses need to understand their information. You, as a data scientist, become the hero by finding hidden patterns and using data to make smart decisions.
Is Data Science Really a Difficult Major?
Let’s be honest, data science isn’t a walk in the park. It throws a blend of complex subjects – statistics, computer science, and industry-specific knowledge – your way. Keeping pace with a rapidly evolving field can feel like a constant marathon.
However, here’s the key takeaway: Difficulty is relative. Students with strong math and programming backgrounds might find the initial climb easier. But even if those aren’t your strengths, with dedication and a willingness to learn, you can excel in data science.
Here’s why data science, despite the challenges, might be the perfect fit for you:
- Lifelong Learning: Data science is a dynamic field that thrives on innovation. You’ll constantly encounter new tools and techniques, keeping your learning journey fresh and exciting.
- Structured Support: Universities are increasingly offering data science programs designed for diverse backgrounds. These programs provide a structured learning environment with hands-on projects and mentorship, making the journey more manageable.
- Real-World Impact: Data science isn’t just about theoretical concepts. You’ll be using your skills to tackle real-world problems and make a tangible difference in various industries.
The answer: Yes, data science can be a demanding major. But with the right approach, the challenges become opportunities for growth. If you’re passionate about learning, solving problems, and making an impact, data science might just be the rewarding adventure you’ve been seeking.
If you’re looking for a comprehensive and structured learning environment with personalized mentorship and hands-on projects, Scaler’s Data Science Course can be a valuable alternative or supplement to traditional university programs.
Is Data Science Hard to Learn for Everyone?
The difficulty of learning data science can vary widely among individuals. Factors such as prior knowledge, educational background, learning style, and problem-solving abilities greatly influence an individual’s learning experience.
For individuals with a solid foundation in mathematics and programming, the learning curve may be less steep. Conversely, those without prior experience in these domains may need to invest additional time and effort to build the necessary skills.
There can be challenges for non-tech folks:
- Math and Coding: Data science involves concepts like statistics and programming languages that might seem unfamiliar at first.
Here’s how you can navigate these challenges:
- Break it Down: Don’t try to swallow the whole data science whale at once! Many online resources offer beginner-friendly tutorials that break down complex topics into manageable steps. There are also courses designed specifically for those with no prior coding experience.
- Focus on the Why: Understanding the
"why"
behind the technical aspects can be a game-changer. Instead of just memorizing code, focus on how it helps you achieve your data science goals. - Find Your Tribe: The data science community such as Kaggle, IBM Data Science community, open data science, etc are welcoming and supportive. Online forums and communities are excellent places to connect with other learners, ask questions, and get valuable advice.
How Long Does It Take to Become a Data Scientist?
The time it takes to become a data scientist can vary considerably depending on several factors, including your prior knowledge, educational background, chosen learning path, and level of commitment.
If you go for a formal degree in data science, it could take you anywhere from one to three years, depending on how deep you want to go. These programs cover a lot of stuff like stats, machine learning, and making data pretty.
But if you’re teaching yourself, it’s more flexible. It could take you a few months to a few years to learn everything you need to know. The key is to stick to a plan, practice with real projects, and really understand what you’re doing.
Data science is always changing, so you’ll need to keep learning even after you finish your studies. Staying up-to-date with the latest tools and tricks is super important to stay competitive.
Talking to other data scientists and joining online communities can also speed up your learning. Discussing ideas, going to workshops, and chatting online can give you new perspectives and chances to improve your skills.
Self-Study vs Formal Education: Can You Learn Data Science on Your Own?
Data science is a rapidly growing field, and many aspiring data scientists wonder: can I learn it on my own? The answer is yes. While formal education offers a structured path, self-study is a viable option for individuals with dedication and the right approach.
Benefits of Self-Study
- Flexibility: Self-study allows you to learn at your own pace, fitting your learning around your schedule and other commitments.
- Cost-Effective: Compared to formal education programs, self-study can be significantly cheaper. There are numerous free online resources available, including tutorials, courses, and bootcamps.
- Focus on Specific Interests: You can tailor your learning journey to your specific interests within data science. This allows you to delve deeper into areas that particularly excite you.
Challenges of Self-Study
- Discipline is Key: Self-study requires a high degree of self-discipline and time management skills. Without a structured program, it’s easy to get sidetracked or lose momentum.
- Building a Foundation: Formal education often provides a strong foundation in math, statistics, and programming, which are crucial for data science. Self-learners may need to invest extra time in building these foundational skills.
- Staying Updated: The data science field is constantly evolving. Self-learners need to be proactive in staying updated with the latest trends and technologies.
Benefits of Formal Education
Formal education programs in data science provide a comprehensive learning experience with several advantages:
- Structured Learning: Formal programs offer a well-defined curriculum that ensures you cover all the essential data science topics, from core mathematics and statistics to programming languages and machine learning algorithms. This structured approach ensures you develop a strong foundation in the fundamentals.
- Career Support: Many universities and colleges offer career services specifically for data science students. These services can help you with resume writing, interview preparation, and job search strategies, giving you a competitive edge in the job market.
- Mentor Support: Formal education allows you to benefit from the guidance and mentorship of experienced instructors. These mentors can provide personalized feedback, answer your questions, and help you navigate the challenges of learning data science.
- Network Building: Reputed courses and programs provide opportunities to connect with peers, professors, and industry professionals. This network can be invaluable for finding internships, securing your first data science role, and staying connected with the latest trends in the field.
If you’re thinking about learning data science, we really suggest going for a formal course. The Scaler Data Science Course is a great pick. It’s like a one-stop shop where you get to learn all the important stuff about data science in a clear, step-by-step way. Plus, you get help with finding a job and advice from people who are already pros in the field. The course always keeps up with what’s new and cool in data science, and you’ll learn from folks who know their stuff inside out. On top of that, you’ll get to connect with other students and experts, which can really help you out in your data science journey.
Conclusion
Though data science may seem challenging at first, it’s essential to differentiate between perception and reality. It’s a multidisciplinary field requiring a strong foundation in math, coding, and statistics.
Think of it as detective work with data. You use these skills to uncover hidden patterns and solve real-world problems. With dedication and the right resources, anyone can learn. Whether through formal education or self-study, the supportive data science community is there to help.
The best part? Data science lets you turn information into something powerful, making a real impact in the world. So, if you’re curious and enjoy challenges, data science could be your perfect adventure!
FAQs
What is the hardest thing about data science?
The hardest thing about data science can vary depending on individual strengths and weaknesses. However, for many people, the most challenging aspects include mastering complex algorithms, staying updated with rapidly evolving technologies, and effectively communicating findings to non-technical stakeholders.
Is data science a hard skill?
Data science is typically considered a hard skill, as it involves technical expertise in areas such as statistics, programming, and data analysis. However, with dedication and practice, anyone can develop proficiency in data science.
Is data science a stressful job?
Data science can be demanding at times, particularly when facing tight deadlines or working on high-stakes projects. However, stress levels can vary depending on factors such as the nature of the work environment, project complexity, and individual coping mechanisms.
Is there a lot of math to learn in Data Science?
Yes, data science involves a significant amount of math, including concepts from statistics, linear algebra, calculus, and probability theory. A solid understanding of these mathematical principles is essential for building and interpreting statistical models, designing algorithms, and making data-driven decisions.
Is data science a high-paying domain?
Yes, data science is considered a high-paying domain. According to recent industry reports, data scientists are among the highest-paid professionals, with salaries ranging from $95,000 to $210,000 annually.
Is data science harder than software engineering?
Data science tends to involve more mathematical and statistical concepts, while software engineering focuses on designing and building software systems. Both fields require problem-solving skills and technical proficiency but may appeal to different interests and strengths.