Data science, with its power to extract meaningful insights from vast amounts of data, is revolutionizing industries on a global scale. India has positioned itself as a key player in this rapidly evolving field. The World Economic Forum predicts that by 2025, data science and AI will create 97 million new jobs globally, and India is poised to play a significant role in this growth.
According to AIMResearch, the Indian data science market is predicted to grow at a Compound Annual Growth Rate (CAGR) of over 33% from 2020 to 2026, indicating a thriving landscape. This growth is driven by increased adoption of data-driven strategies across sectors like healthcare, finance, e-commerce, and manufacturing. In their quest to remain competitive in the digital era, businesses are making substantial investments not only in recruiting data science talent but also in implementing data analytics tools and fostering data literacy among their existing workforce.
This surge in demand translates to a promising future for data science professionals. The data science field offers an array of career paths, including roles as data analysts, data scientists, machine learning engineers, and AI specialists. With the right skills and expertise, you can unlock a world of opportunities in this lucrative and impactful field.
Ready to embark on a career in data science? Explore Scaler’s Data Science course and equip yourself with the skills needed to thrive in this dynamic industry!
Current State of Data Science
Data science is no longer a futuristic concept; it’s a driving force in today’s world, revolutionizing industries and shaping how we live and work. Its applications span a vast array of fields, influencing everything from personalized recommendations to medical diagnoses and autonomous vehicles. Let’s explore some of the most notable achievements and breakthroughs that have solidified data science’s position as a game-changer:
Present Applications
Data science has seamlessly integrated into every facet of our lives, often without our explicit awareness. Here are a few exemplary instances of its current applications:
- Personalized Recommendations: Major streaming platforms like Netflix and Spotify harness the power of data science algorithms to analyze user’s viewing and listening habits, thereby customizing recommendations to cater to individual tastes.
- Fraud Detection: Banks and financial institutions employ machine learning models to detect fraudulent transactions in real time, protecting consumers and preventing financial losses.
- Medical Diagnosis: Data science is revolutionizing healthcare by enabling faster and more accurate diagnoses. Machine learning algorithms can analyze medical images, such as X-rays and scans, to identify tumors, abnormalities, and potential health risks.
- Self-Driving Cars: Autonomous vehicles depend on an intricate network of sensors and data science algorithms. These technological marvels navigate roads, detect obstacles, and make instantaneous decisions, setting the stage for a safer and more efficient future of transportation.
- Customer Service: AI-powered chatbots and virtual assistants, backed by data science, are being increasingly deployed to provide instant customer support. They efficiently answer queries and resolve issues, enhancing customer service standards and efficiency.
- Supply Chain Optimization: Data science helps businesses optimize their supply chains by forecasting demand, managing inventory levels, and improving logistics, leading to reduced costs and faster delivery times.
Key Achievements and Breakthroughs
Data science has been at the forefront of several groundbreaking advancements in recent years, including:
- AlphaFold (DeepMind): AlphaFold, an AI system developed by DeepMind, has transformed the field of protein folding prediction – a complex problem that has stymied scientists for decades. Its accurate predictions have the potential to accelerate drug discovery and revolutionize medicine.
- GPT-3 (OpenAI): GPT-3, a cutting-edge language model from OpenAI, can generate text that remarkably resembles human writing. It can translate languages, craft various types of creative content, and provide informative answers to queries, showcasing the diverse capabilities of AI in language processing.
- Image Generation (DALL-E 2): OpenAI’s DALL-E 2 can create realistic images and art from a description in natural language, showcasing the power of AI in creative fields.
These examples are just the tip of the iceberg when it comes to the impact of data science. The field is constantly evolving, with new applications and breakthroughs emerging regularly. As data continues to proliferate, data science will play an increasingly crucial role in shaping our future.
Emerging Trends in Data Science
The realm of data science is dynamic, characterized by continuous evolution, with innovative trends and technologies poised to transform the field. Let’s explore some of the most promising developments set to redefine the future of data science:
- AI and Machine Learning Integration: The integration of artificial intelligence and machine learning is becoming increasingly sophisticated, enabling data scientists to develop more powerful and accurate models. This collaboration is catalyzing breakthroughs in natural language processing, computer vision, and predictive analytics, reshaping industries from healthcare to finance and e-commerce.
- Edge Computing and IoT: The proliferation of Internet of Things (IoT) devices is generating massive amounts of data at the network’s edge. Edge computing, which processes data closer to its source, is emerging as a key trend, enabling real-time analytics and decision-making for applications like smart cities, industrial automation, and autonomous vehicles.
- Automated Machine Learning (AutoML): AutoML is democratizing data science by automating labor-intensive tasks such as data preprocessing, feature engineering, and model selection. This allows non-experts to leverage machine learning capabilities and focus on higher-level tasks like problem definition and solution interpretation.
- Explainable AI (XAI): As AI models become more complex, understanding their decision-making processes becomes crucial, especially in high-stakes applications like healthcare and finance. Explainable AI aims to make these black-box models more transparent and interpretable, providing insights into how they arrive at their predictions and ensuring fairness and accountability.
- DataOps and MLOps: Analogous to how DevOps revolutionized software development, DataOps and MLOps are altering the way data science and machine learning projects are managed. These practices emphasize collaboration, automation, and continuous integration/continuous delivery (CI/CD) to streamline the entire data science lifecycle, from data ingestion and preparation to model deployment and monitoring.
These emerging trends represent a paradigm shift in data science, promising to accelerate innovation, democratize access to powerful tools, and unlock new possibilities for solving complex problems across various domains. By staying abreast of these trends and embracing their potential, data scientists can position themselves at the forefront of this exciting and rapidly evolving field.
Industry-Specific Trends
Data science and machine learning, once the realm of tech giants and research labs, are now transforming industries across the spectrum, heralding a new era of innovation and efficiency. Let’s delve into some of the most significant trends in different sectors:
1. Healthcare
- Personalized Medicine: Data science is paving the way for personalized medicine by analyzing individual patient data, including genetics, lifestyle, and medical history, to tailor treatment plans and optimize outcomes.
- Predictive Analytics: Machine learning algorithms can analyze vast amounts of patient data to predict disease risk, identify potential complications, and recommend preventive measures.
2. Finance
- Fraud Detection: AI-powered systems are becoming increasingly sophisticated in detecting fraudulent activities like credit card fraud and money laundering. They can analyze transaction patterns and identify anomalies in real time, protecting both consumers and financial institutions.
- Algorithmic Trading: Machine learning algorithms are used to analyze market data, identify trends, and execute trades automatically. These algorithms can process vast amounts of information and make decisions at lightning speed, leading to increased efficiency and potential profits.
3. Retail and E-commerce
- Customer Personalization: Recommendation engines leverage data science to analyze customer behavior and preferences, offering tailored product suggestions and personalized shopping experiences. This drives engagement, boosts sales, and enhances customer loyalty.
- Inventory Management: Data science helps retailers optimize inventory levels by forecasting demand, predicting stockouts, and automating replenishment processes. This minimizes waste, reduces costs, and ensures that products are available when customers need them.
4. Cybersecurity
- Threat Detection: Machine learning algorithms can analyze network traffic and system logs to identify patterns that indicate cyberattacks, allowing for faster detection and response to security threats.
- Risk Management: Data science models can assess risk profiles and predict potential vulnerabilities, helping organizations proactively address security issues before they escalate.
5. Transportation
- Autonomous Vehicles: Self-driving cars and trucks are becoming a reality, thanks to advancements in data science and machine learning. These vehicles use sensors, cameras, and AI algorithms to navigate roads, detect obstacles, and make driving decisions.
- Route Optimization: Data science is used to optimize delivery routes for logistics companies, considering factors like traffic, weather, and fuel consumption. This reduces costs, saves time, and minimizes environmental impact.
6. Education
- Adaptive Learning Technologies: Data-driven platforms personalize learning experiences for students, tailoring content and pace to their individual needs and progress. This leads to improved learning outcomes and student engagement.
- Student Performance Prediction: Machine learning models can analyze student data to predict their academic performance and identify those who may need additional support. This allows for early intervention and targeted interventions to improve student success.
Data Science Job Trends in 2024
The increasing recognition of the transformative power of data-driven insights by businesses across industries has led to a surge in demand for skilled data scientists. Let’s take a closer look at the job trends shaping the field in 2024:
1. Unwavering Demand for Data Scientists
- Exponential Growth: The demand for data scientists is experiencing exponential growth, with projections indicating a significant increase in job openings in the coming years. The World Economic Forum predicts that by 2025, data science and AI will create 97 million new jobs globally, further highlighting the immense potential of this field.
- Industry-Agnostic Demand: Data science skills are no longer confined to the tech sector. Industries like healthcare, finance, retail, manufacturing, and even government are actively seeking data scientists to leverage data for innovation, efficiency, and growth.
- Competitive Salaries: The high demand for data science talent translates to competitive salaries, with experienced professionals commanding lucrative compensation packages.
2. Rise of New Job Roles and Specializations
- AI and ML Specialists: As artificial intelligence and machine learning become more sophisticated, specialized roles like AI engineers, machine learning researchers, and NLP experts are in high demand. These professionals focus on developing cutting-edge algorithms and models that can automate tasks, make predictions, and drive innovation.
- Data Engineers: The growing volume and complexity of data require skilled data engineers who can design, build, and maintain robust data pipelines and infrastructure. These professionals ensure that data is accessible, reliable, and ready for analysis, making them essential members of data science teams.
- Data Product Managers: Bridging the gap between technical and business teams, data product managers define the vision, strategy, and roadmap for data-driven products and services. They ensure that data science initiatives align with business goals and deliver value to customers.
3. Skills in High Demand
- Machine Learning and Deep Learning: Expertise in these areas, including knowledge of popular frameworks like TensorFlow and PyTorch, is highly sought after. Employers are looking for data scientists who can build and deploy complex models to solve real-world problems.
- Big Data Technologies: With the exponential growth of data, skills in big data technologies like Hadoop and Spark are increasingly valuable. Data scientists who can efficiently process and analyze massive datasets are in high demand.
- Cloud Computing: As more businesses move their data and applications to the cloud, knowledge of cloud platforms like AWS, Azure, and GCP is becoming essential for data scientists.
- Domain Expertise: In addition to technical skills, domain expertise in areas like healthcare, finance, or marketing can give you a competitive edge. Employers value data scientists who understand the nuances of their industry and can apply data-driven insights to solve specific business challenges.
The Role of Data Science in Shaping the Future
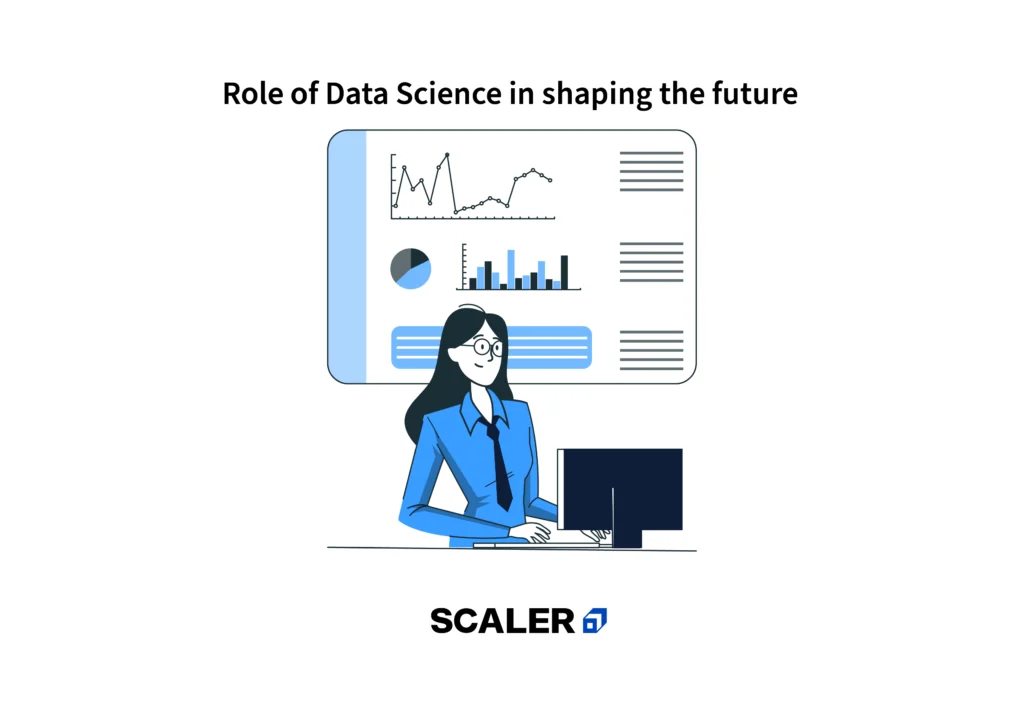
Data science is a force that is changing industries, spurring innovation, and completely altering the way we live and work. It is more than just a tool. As we move further into the digital age, data science’s influence on business decision-making, technological advancements, and ethical considerations is only set to grow.
1. Revolutionizing Business Decision-Making:
Businesses are using data science to make more informed decisions based on data. By analyzing vast amounts of information, organizations can gain valuable insights into customer behaviour, market trends, and operational efficiency. This enables them to identify new opportunities, optimize processes, personalize experiences, and ultimately, achieve sustainable growth and profitability. Whether it’s predicting customer churn, optimizing pricing strategies, or identifying the next big product trend, data science is transforming how businesses operate and compete in the market.
2. Accelerating Technological Advancements:
Data science is at the heart of some of the most groundbreaking technological advancements of our time. Artificial intelligence, machine learning, and deep learning are all fueled by data, enabling the development of intelligent systems that can learn, adapt, and perform complex tasks. From self-driving cars and medical diagnosis tools to personalized recommendations and fraud detection systems, data science is pushing the boundaries of what’s possible and shaping the future of technology.
3. Navigating Ethical Considerations and Challenges:
As data science becomes increasingly pervasive, ethical considerations and challenges are coming to the forefront. Data privacy, algorithmic bias, and the potential for misuse of data are concerns that need to be addressed. Data scientists and organizations must ensure that data is collected and used responsibly, with transparency and accountability. Striking the right balance between innovation and ethical practices is crucial for building trust and ensuring the responsible and beneficial use of data for societal progress.
In conclusion, data science is a transformative force that is reshaping our world in profound ways. Its impact on business, technology, and society is undeniable. As we move into the future, the role of data science will only become more significant, and its potential for innovation and progress is limitless.
Ready to dive into the transformative world of data science? Explore Scaler’s Data Science course and equip yourself with the skills to lead in this evolving field!
Skills Required for Future Data Scientists
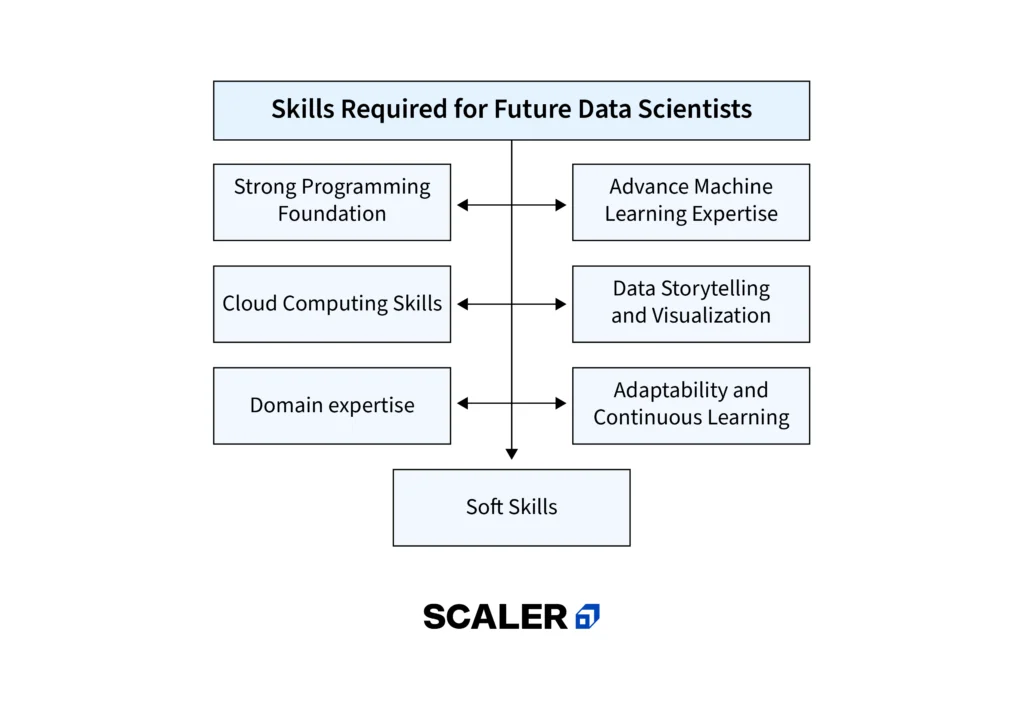
Data scientists need to have a broad range of flexible skills in order to stay on top of the ever-changing and dynamic field of data science. Here are the key skills that will be in high demand for future data scientists:
- Strong Programming Foundation: Proficiency in programming languages like Python, R, or Julia is essential for data manipulation, analysis, and model building. Comprehending foundational ideas such as object-oriented programming, data structures, and algorithms is also essential.
- Advanced Machine Learning Expertise: As machine learning continues to advance, data scientists need to be well-versed in a variety of algorithms, including deep learning techniques like neural networks. This includes understanding how to train and evaluate models, optimize hyperparameters, and deploy models in production environments.
- Cloud Computing Skills: The cloud is increasingly becoming the backbone of data science infrastructure. Acquiring knowledge of cloud platforms such as AWS, Azure, or Google Cloud Platform (GCP) is essential for effectively managing and evaluating sizable datasets, developing models, and implementing massive applications.
- Data Storytelling and Visualization: Data scientists need to be effective communicators who can translate complex data into actionable insights. Proficiency in data visualization, with tools such as Tableau or Power BI, is imperative for crafting captivating dashboards and reports that narrate a story.
- Domain expertise: While technical proficiency is necessary, it is just as important to comprehend the particular sector or field you are working in. Domain expertise allows you to ask the right questions, interpret data in context, and tailor your solutions to address specific business needs.
- Adaptability and Continuous Learning: Data science is a rapidly evolving field, with new technologies and tools emerging constantly. Future data scientists must embrace a lifelong learning mindset, stay up-to-date with the latest trends, and continuously upskill to remain competitive.
- Soft Skills: In addition to the above technical expertise, data scientists need strong soft skills like critical thinking, problem-solving, communication, and collaboration. These skills are essential for working effectively in teams, understanding business objectives, and presenting findings to stakeholders.
In order to succeed in 2024 and beyond, aspiring data scientists should acquire this extensive skill set. The future of data science is bright, and those who embrace the challenge of continuous learning and skill development will be well-prepared to thrive in this exciting and impactful field.
Educational Pathways
Continuous learning and a solid educational foundation are essential for a successful career in data science. Whether you’re just starting or looking to upskill, various educational pathways can equip you with the knowledge and skills you need to thrive in this exciting field.
Recommended Courses and Certifications
1. Bachelor’s or Master’s Degrees
A strong theoretical foundation can be obtained with a degree in a quantitative field like data science, computer science, statistics, or mathematics. Master’s programs offer a deeper dive into specialized areas like machine learning, big data, and AI.
2. Scaler Data Science and Machine Learning Course
The demanding, industry-tested curriculum of this extensive program, created by seasoned experts from top tech firms, sets it apart. It covers a wide range of topics, from Python and SQL fundamentals to advanced machine learning algorithms and big data technologies. Enroll Now!
The key features and benefits are:
- 1:1 Mentorship: Receive personalized guidance and support from industry experts who have worked on real-world projects, ensuring you get the most out of your learning experience.
- Live Classes and Doubt Resolution: Interact with instructors and peers in real time, ask questions, and get your doubts clarified, creating a collaborative learning environment.
- Real-World Projects: Gain practical experience by working on real-world projects that simulate the challenges faced by data scientists in the industry. Build a portfolio to showcase your skills to potential employers.
- Career Support: Scaler’s dedicated career services team offers resume building workshops, mock interviews, and networking opportunities to help you land your dream job in data science.
3. Certifications
Industry-recognized certifications like the Google Data Analytics Professional Certificate or Microsoft Certified: Azure Data Scientist Associate validate your skills and enhance your resume.
4. Online Courses
Platforms like edX, and Udemy offer a wealth of data science courses from top universities and institutions. These courses provide flexibility and affordability, allowing you to learn at your own pace and choose topics that align with your interests.
5. Bootcamps
If you’re looking for an intensive, immersive, and career-focused learning experience, bootcamps can be a great option. They offer structured curricula, hands-on projects, and career support to fast-track your journey into data science.
6. Certifications
Industry-recognized certifications like the Google Data Analytics Professional Certificate or Microsoft Certified: Azure Data Scientist Associate validates your skills and demonstrates your expertise to potential employers.
Importance of Continuous Learning
Data science is a rapidly evolving field, with new technologies and techniques emerging constantly. To stay ahead of the curve, continuous learning is essential. This involves staying updated with the latest industry trends, reading research papers, experimenting with new tools, and participating in online communities and forums.
Career Growth Opportunities
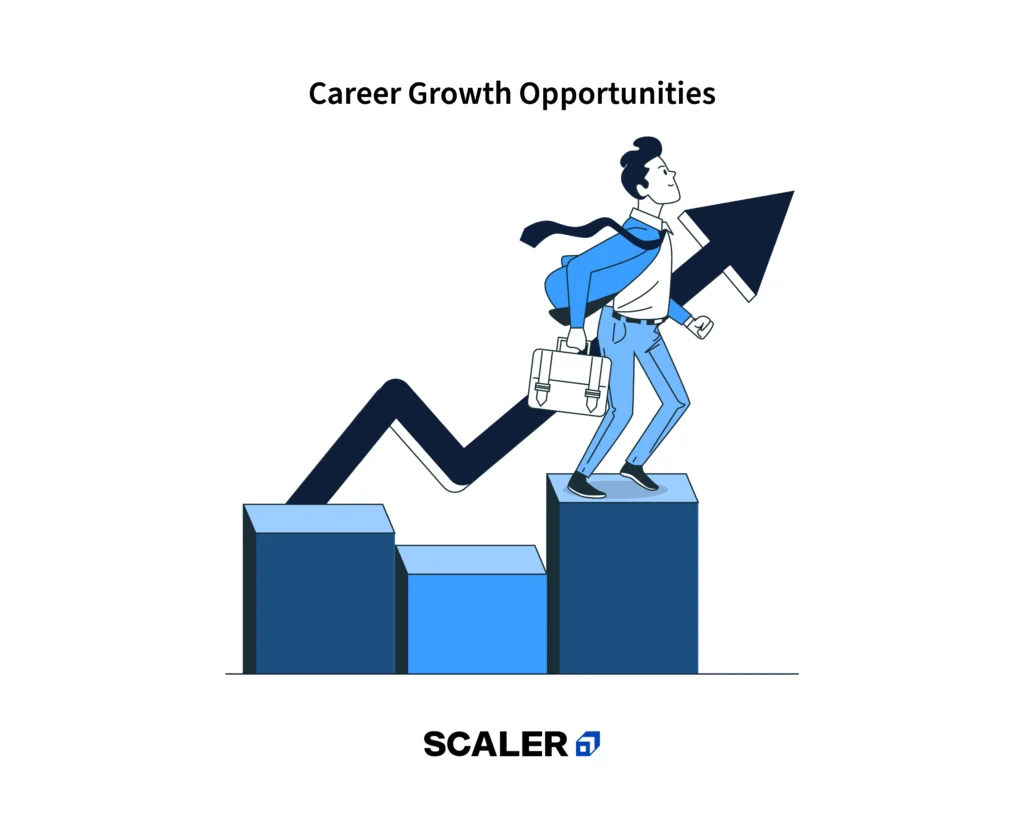
There are many different career paths in the data science and artificial intelligence fields, and as you gain experience and expertise, you can grow and specialize. Here’s a glimpse into the potential career trajectory and emerging roles in these exciting domains:
Entry-Level Roles
- Data Analyst: Starting as a data analyst allows you to gain hands-on experience in data cleaning, analysis, and visualization. Working with programs like SQL, Excel, and Tableau, you will extract insights from data and effectively convey them to stakeholders.
- Junior Data Scientist/AI Engineer: In these roles, you’ll typically work under the supervision of senior professionals, gaining exposure to various aspects of data science and AI projects. As you gradually gain knowledge and autonomy, you will participate in data preparation and collection, model building, and implementation.
Mid-Level Roles
- Data Scientist: As you progress in your career, you can move into a full-fledged data scientist role, where you’ll be responsible for designing and executing end-to-end data science projects. This includes formulating problem statements, gathering and evaluating information, creating models, and sharing conclusions with interested parties.
- Senior Data Analyst/AI Engineer: With a few years of experience, you can take on senior roles with more responsibility and leadership. To promote data-driven decision-making, you might oversee data analysis projects, coach more junior team members, and work with cross-functional teams.
Senior-Level Roles
- Lead/Principal Data Scientist/AI Engineer: At the senior level, you’ll be responsible for leading data science or AI teams, overseeing complex projects, and providing technical guidance and mentorship. It will be expected of you to create and carry out strategic data initiatives that complement the objectives of the company.
- Data Science Manager/Director: These leadership roles involve managing data science teams, setting strategic direction, and ensuring the successful execution of data-driven projects. Allocating resources, creating a budget, and working with other departments to use data to impact the business will all fall under your purview.
Emerging Roles
- AI Ethics Specialist: As AI becomes more prevalent, ethical considerations are becoming increasingly important. In order to ensure justice, accountability, and transparency in the development and application of AI systems, experts in AI ethics evaluate the ethical implications of these systems.
- Data Privacy Officer: With the growing importance of data privacy regulations like GDPR and CCPA, data privacy officers play a crucial role in ensuring that organizations comply with these laws and protect sensitive data.
Case Studies and Examples
To truly grasp the impact of data science, let’s examine real-world examples of how it’s being used to solve complex problems and drive innovation:
1. Netflix’s Recommendation Engine
- The Problem: How to keep users engaged and increase watch time by suggesting relevant content.
- Data Science Solution: Netflix employs machine learning algorithms to analyze massive amounts of viewer data, including watch history, ratings, and search behavior. This data fuels their recommendation engine, which suggests personalized content for each user.
- Outcome: Netflix estimates that their recommendation system saves the company over $1 billion annually by reducing churn and increasing customer retention.
2. Target’s Pregnancy Prediction Model
- The Problem: How to identify and target expectant mothers with relevant marketing campaigns.
- Data Science Solution: Target’s data scientists developed a predictive model that analyzed customer purchase history to identify patterns associated with pregnancy. The model could predict which customers were likely pregnant, even before they announced it.
- Outcome: This targeted marketing strategy led to a significant increase in sales of baby-related products, demonstrating the power of data-driven insights in retail.
3. Amazon’s Anticipatory Shipping
- The Problem: How to reduce delivery times and improve customer satisfaction.
- Data Science Solution: Amazon leverages vast amounts of customer data to predict what products customers are likely to buy. In some cases, they even ship these products to local warehouses before an order is placed, significantly reducing delivery times.
- Outcome: Amazon’s anticipatory shipping has been a major success, enhancing customer experience and driving brand loyalty.
4. Google’s Waymo: Self-Driving Cars
- The Problem: How to develop safe and reliable autonomous vehicles.
- Data Science Solution: Waymo, Google’s self-driving car project, uses a combination of data science, machine learning, and sensor technology. Their vehicles collect and analyze data from their surroundings to learn how to navigate roads, detect obstacles, and make driving decisions.
- Outcome: Waymo has made significant progress in developing self-driving technology, with its vehicles logging millions of miles of real-world testing and demonstrating the potential to revolutionize transportation.
5. IBM Watson: Revolutionizing Healthcare
- The Problem: How to improve medical diagnosis and treatment decisions.
- Data Science Solution: IBM Watson, an AI-powered platform, analyzes vast amounts of medical data, including patient records, clinical trials, and research papers, to assist doctors in making diagnoses and treatment recommendations.
- Outcome: Watson has been used to diagnose rare diseases, personalize cancer treatments, and even assist in surgical procedures, showcasing the potential of AI in healthcare.
These case studies illustrate the diverse and impactful ways data science is transforming industries. From personalized entertainment to life-saving medical treatments and innovative transportation solutions, data science is shaping a smarter, more efficient, and more personalized future.
Conclusion
Data science is rapidly evolving, with exciting trends shaping its future landscape. We can expect AI and machine learning to become even more integrated into our lives, with applications ranging from personalized medicine to self-driving cars.
Data scientists will play a crucial role in developing these innovative solutions. To thrive in this field, they’ll need to continuously learn and adapt, staying ahead of the curve by mastering new technologies like edge computing, automated machine learning, and explainable AI.
The field of data science has a bright future ahead of it. As data continues to grow in volume and complexity, so too will the opportunities for data-driven innovation and problem-solving. Whether you are a seasoned professional or you are just getting started in data science, embracing these trends and developing your skills will make sure you are ready to handle the fascinating and constantly shifting terrain of this dynamic field.
FAQs
What are the future trends in data science?
Data science is rapidly evolving, with emerging trends like explainable AI (making AI decisions transparent), automated machine learning (democratizing data science), and the rise of data-centric AI (prioritizing data quality). Other key trends include edge computing for real-time analytics and DataOps for streamlined workflows.
How will data science impact different industries?
Data science is already transforming industries by enabling personalized customer experiences, improving healthcare outcomes, optimizing supply chains, and enhancing financial decision-making. In the future, its impact will deepen as we see advancements in areas like autonomous vehicles, smart cities, and precision medicine.
What skills will be in demand for future data scientists?
Future data scientists will need a combination of technical skills (like machine learning, big data, and cloud computing) and soft skills (like communication, problem-solving, and domain expertise). Adaptability and a willingness to learn new technologies will also be crucial.
What are the new job roles emerging in data science?
As the field evolves, we’re seeing the rise of specialized roles like AI Ethics Specialist, Data Privacy Officer, and Data Product Manager. These roles address the ethical implications of AI, ensure data privacy compliance, and bridge the gap between data science and business strategy.
How can one stay updated with the latest trends in data science?
To stay ahead of the curve, engage with the data science community by attending conferences, workshops, and webinars. Follow industry blogs, subscribe to newsletters, and participate in online forums to learn about new developments, tools, and techniques.