Data Science Vs Data Analytics
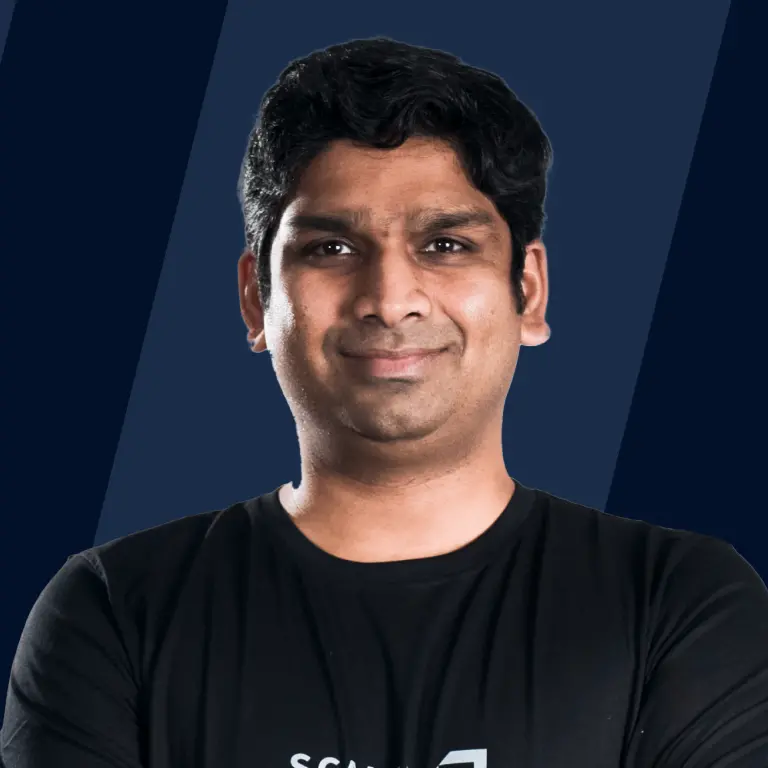
In the ever-evolving world of data, understanding the nuances between data science and analytics is crucial for leveraging data effectively. This article delves into these distinct yet interconnected fields, shedding light on their roles, tools, and applications.
What is Data Science?
Data science is an interdisciplinary field that leverages a wide array of statistical, analytical, and programming skills to collect, clean, and analyze large sets of data, drawing actionable insights and solutions to complex problems. It encapsulates a broader perspective on data manipulation and interpretation, encompassing predictive analytics, machine learning, and data mining to forecast future trends and behaviors. The relationship between data science and analytics is foundational to the digital age, marking a pivotal aspect of how organizations strategize and make informed decisions. The distinction between data science and data analytics is crucial, as it highlights the proactive, exploratory nature of data science compared to the more focused, immediate objectives of data analytics.
In the realm of data science and analytics, professionals employ advanced computational tools and algorithms to process and analyze data, aiming to derive predictive insights and innovative solutions. This contrasts with data analytics, which primarily focuses on extracting actionable insights from historical data. Understanding the difference between data science and data analytics is key for businesses and organizations aiming to leverage data effectively, ensuring they adopt the right approach and tools for their specific needs. While data analytics offers a snapshot of the past and present, data science looks forward, paving the way for breakthrough innovations and strategic foresight in an increasingly data-driven world.
What is Data Analytics?
Data Analytics is a subset within the broader field of data science and analytics, focusing on processing and analyzing datasets to draw conclusions, identify trends, and support decision-making processes. It employs statistical techniques and software tools to interpret, visualize, and communicate data findings, catering primarily to operational improvements and efficiency. The practice is instrumental in various industries, including healthcare, finance, and marketing, where making data-driven decisions is pivotal. The difference between data science and data analytics lies mainly in the scope and end goals; data analytics is more about answering specific questions and solving problems based on historical data insights.
Data Science Vs Data Analytics
Understanding the distinction between data science and data analytics is crucial for leveraging their potential in the digital landscape. While both fields work with data, their approaches, methodologies, tools, and ultimate goals differ significantly, offering unique insights and solutions to data-related challenges.
Aspect | Data Science | Data Analytics |
---|---|---|
Objective | To explore and analyze complex data to discover underlying patterns, predict future trends, and derive actionable insights. | To process and analyze data to find answers to specific questions, often with a focus on understanding past or current data trends. |
Scope | Broad, aiming to build models and systems for predicting future events or understanding complex behaviors. | Narrower, focusing on analyzing historical data to improve current processes or make informed decisions. |
Techniques and Tools | Machine learning, predictive modeling, statistics, natural language processing, big data technologies. Common tools include Python, R, TensorFlow, and Apache Hadoop. | Statistical analysis, data mining, data cleaning, and visualization. Common tools include SQL, Excel, Tableau, and Power BI. |
Applications | Broad applications across sectors like tech, finance, healthcare, e-commerce, and more for developing AI systems, recommendation engines, and predictive models. | Specific applications in business intelligence, financial analysis, marketing analytics, and operational efficiency. |
Outcome | Develops new insights and predictive models that can lead to innovative products and solutions. | Provides concrete data insights that inform strategic decisions and improve operational efficiencies. |
Focus | Investigative, looking to ask the right questions and find new opportunities within vast datasets. | Solution-oriented, aimed at solving specific, predefined problems or answering questions based on data analysis. |
FAQs
Q. What is the main goal difference between data science and data analytics?
A. Data science aims to create models and predict future trends, while data analytics focuses on analyzing past data to make informed decisions.
Q. Can you transition from data analytics to data science?
A. Yes, many professionals transition from data analytics to data science as they gain more experience in statistical methods, programming, and machine learning.
Q. Which is more suitable for small businesses, data science or data analytics?
A. Small businesses might benefit more from data analytics initially, as it helps in understanding market trends and customer behaviors to make immediate decisions.
Q. Do data science and data analytics require different skill sets?
A. While both fields overlap, data science typically requires deeper knowledge in machine learning, programming, and advanced statistical methods, whereas data analytics focuses more on statistical analysis and interpretation of data.
Related Topics
Explore the intricate world of data through these related topics, offering insights into the dynamic roles, methodologies, and applications within the fields of data science and analytics.
-
Data Analyst vs Data Scientist:
Dive deeper into the distinctions and synergies between these two pivotal roles in the data realm. Explore More -
Machine Learning Vs Artificial Intelligence:
Understand the relationship and differences between these two groundbreaking technologies shaping our future. Read More -
Big Data:
Concepts and Applications: Delve into the world of big data, exploring its concepts, tools, and how it's transforming industries across the globe. Learn More
Conclusion
- Understanding the nuanced differences between data science and data analytics is crucial for effectively leveraging data in decision-making processes. Both fields, while interconnected, serve distinct purposes within the realm of data exploration and analysis.
- A solid foundation in both data science and data analytics provides a versatile skill set, but specialization requires focusing on the specific tools, techniques, and objectives unique to each field.
- Businesses and organizations can harness the power of data science for predictive modeling and innovation, while data analytics is indispensable for operational insights and immediate decision-making.
- The distinction between data science and data analytics also mirrors career pathways, where individuals can start with data analytics and evolve into data science roles as they gain expertise in machine learning, advanced analytics, and programming.
- As data continues to drive decision-making processes across industries, the strategic application of data science and data analytics will remain pivotal in uncovering trends, informing strategies, and fostering innovation.